The Future of AI: How Vector Databases Shape Data Intelligence

By Edmond Leung
Published on May 3, 2024
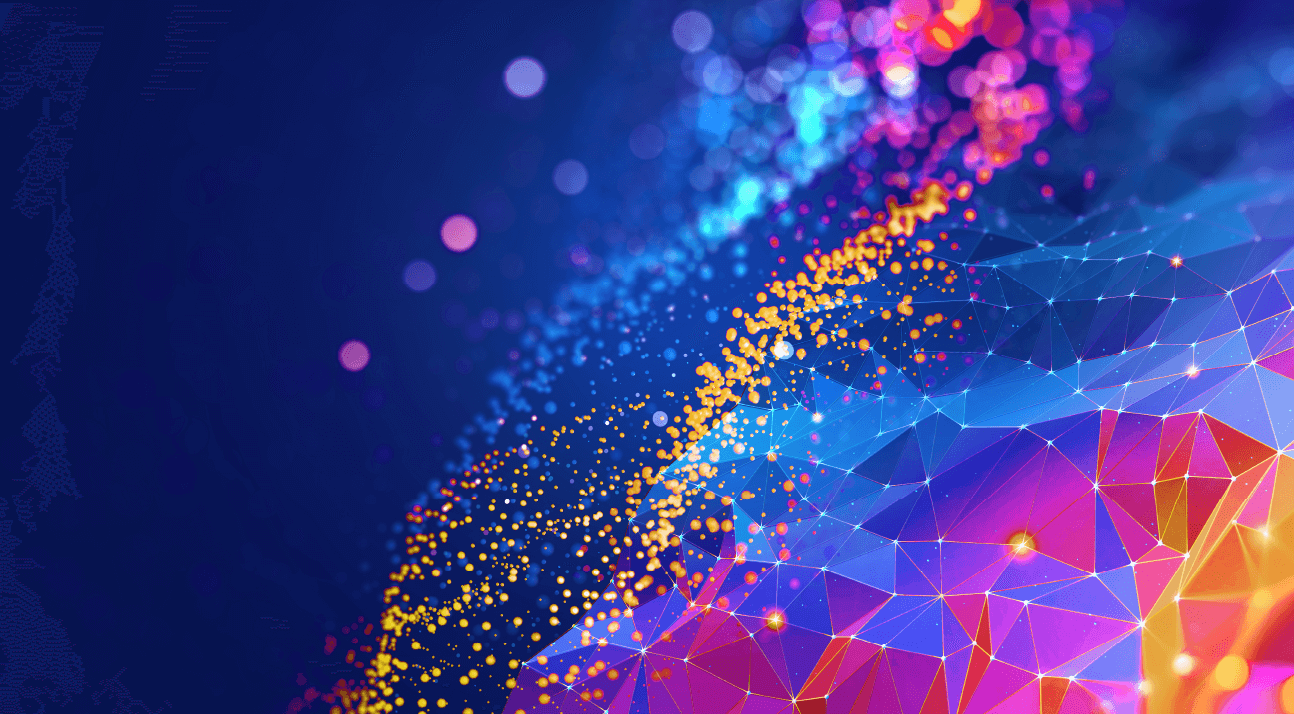
In the world of AI, the emergence of vector databases has been nothing short of revolutionary. Vector databases represent a paradigm shift in data intelligence. Powerful Large Language Models (LLMs) can translate or ‘encode’ data, such as the meanings of text paragraphs or the essence of images, into vectors and store them in vector databases. With well-defined mathematics from vector theories, vector databases help users find paragraphs that are similar in meaning or images that are similar in color, shape, texture, and composition.
Pinecone is a vector database company that has rapidly gained recent attention due to its ability to add context to large language models. To learn more about the potential of vector databases, Satyen Sangani, CEO and co-founder of Alation, sat down with Edo Liberty, CEO and co-founder of Pinecone. Here are the key takeaways from that conversation.
Vector databases are key to semantic search
At the heart of vector databases lies the capability to process text and generate semantic representations of content more efficiently than ever before. “Semantic search was, in some sense, a holy grail for 30 years,” Liberty says. “Now you can put these systems on steroids, and companies like Notion, Gong, and many others have built question-answering and analogy discovery and so on, for their own tens of thousands of customers… That's possible today, which was not possible even like two or three years ago,” he points out.
Semantic search has a broad range of applications
Due to their versatility, vector databases serve a broad spectrum of use cases beyond augmenting foundational AI models. Liberty emphasizes, "Search and semantic search, the ability to really search for things by meaning and by analogy and by correlation, is a very powerful thing. It's used for shopping recommendations, and legal discovery, and anomaly detection in financial data, and fraud detection, and spam filtering, and a million different platforms that acquire this foundational layer.” In this way, the versatility of vector databases has made them indispensable across various domains.
Vector databases for data intelligence
Vector databases represent a paradigm shift in data intelligence, offering new foundational capabilities in semantic search, knowledge retrieval, and reasoning. As organizations continue to harness the power of AI and machine learning, understanding and leveraging vector databases like Pinecone will be essential for driving innovation and unlocking new possibilities in data-driven decision-making. With careful consideration of ethical and regulatory concerns, vector databases have the potential to revolutionize the way we interact with and derive insights from data.
Here are just a few examples of the potential benefits of vector databases for data intelligence platforms such as Alation:
Harness the power of metadata
Vector databases power semantic search, the ability to search by the meaning of a search string, leading to a significant jump in search accuracy.
In this way, the data catalog presents a prime use case for vector databases. Consider a user who seeks to use semantic search on their catalog's metadata. They could use trained models to vectorize both the content and the search questions. The model would then compare the output vectors to find the nearest matches for search results. The vectors in this case are simply different representations of the cataloged content. Depending on the goals of the data science teams, leaders could use different models to vectorize a subset of the content for their own ML purposes.
Superior semantic search
It’s easy to find the enterprise data you need if you know how it’s labeled. But what if you don’t know? This is where semantic search becomes critical. This capability empowers Alation users to search using natural language, so that relevant content is ranked at the top of the results list.
Today, sophisticated semantic search in the data catalog casts a wider net of meaning to return relevant results. Let's take the example of an analyst working at a life sciences company. If they search "Heart disease fatality rate" within Alation, the search results may not match this precise phrase. However, because semantic search understands the meaning of the phrase, they will see relevant results, such as "Cardiovascular death rate." This empowers users to quickly locate information even if they do not know the specific terminology used to describe the relevant data assets across the data intelligence platform.
Teaching AI to reason is the next frontier
We all know that inaccuracy is a core challenge of AI today. So how can AI model builders push their LLMs to be more accurate? Liberty points to “RAG, or Retrieval Augmented Generation” as a “necessary component.” AWS defines RAG as the process of optimizing the output of a large language model so it references an authoritative source external to its training data before generating a response.
Liberty explains, "It's the ability to go and fetch relevant information in real time and for the foundational model to actually respond intelligently with data." By storing and efficiently retrieving relevant information, vector databases can empower the leaders building LLMs and AI tools to craft solutions that respond intelligently (with unique organizational context) to queries. This emerging ability of AI to “reason” by referencing trusted sources represents massive potential for the next frontier of AI initiatives.
Trust is often the largest barrier for enterprises to accept AI-generated responses. And trust comes in two forms. First, is the content source informing the LLM’s response trusted? Second, are the users allowed to see the content sources from which the response is derived? In other words, are those users entrusted or permitted to access the underlying content informing the generated responses?
A data intelligence platform can address both of these questions to create trust in the generated responses. The platform can show users the sources utilized by the LLM, giving users the option to verify authority. The platform itself can also offer role-based access control to regulate individual users’ access to the responses, ensuring unpermitted users will not see the response by accident.
Regulating AI demands a multidisciplinary approach
Throughout human history, technology has typically outpaced the legislation that regulates it. And while the potential of vector databases is immense, there are ethical and regulatory considerations that need to be addressed. Liberty acknowledges the importance of regulation focusing on use cases rather than technology itself, stating, "I am concerned when regulation specifies how a technology can be used rather than what it can be used for." To ensure responsible deployment of vector databases, Liberty favors a collaborative approach involving technologists, policymakers, and industry stakeholders who can balance theory with practice.
Curious to see how you can leverage Alation for groundbreaking AI use cases? Schedule a personalized demo with us today.
- Vector databases are key to semantic search
- Semantic search has a broad range of applications
- Vector databases for data intelligence
- Teaching AI to reason is the next frontier
- Regulating AI demands a multidisciplinary approach