A Data Analyst’s Guide to the Data Catalog
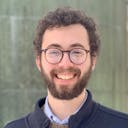
By Joe Hilleary
Published on May 17, 2022
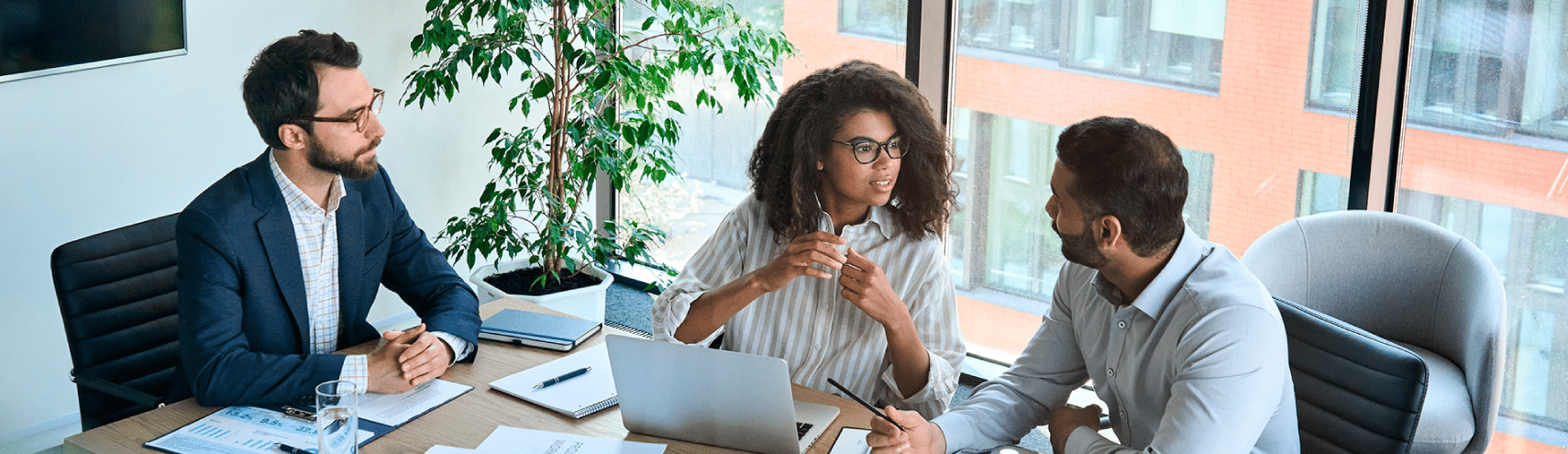
The value of an analytics program stems from its ability to answer business questions. As the role in charge of crafting those answers, it should come as no surprise that the most important persona in any analytics program is the analyst.
Without the analyst, there’s no analytics. Folks can talk all day about automated insights, but answering ad hoc business questions still relies on human beings who understand the business context and how their bosses communicate. And today, it’s much harder to scale a person than a machine learning model. As a result, providing analysts with tools that increase their efficiency is critical to helping them do more work with less effort. An enterprise data catalog is one such key asset.
The Data Analyst Workflow
The workflow of a data analyst consists of four key stages:
Discovery
Preparation
Analysis
Reporting
Each stage has its own set of tasks and corresponding toolkit. (See figure 1.)
When a businessperson poses a question to an analyst, the analyst starts by trying to find the right data. This means not only locating potentially relevant tables, but also vetting them to ensure they are trustworthy. The analyst must determine what insights a table might provide, the accuracy of the data, and the meaning and original context of the information it communicates.
Once the analyst knows what data they can use, they must query it, prepare it, and perform the actual analysis. In the final phase (reporting), the analyst communicates the answer or insight they uncovered back to the original requestor and anyone else who might benefit from the information.
A data catalog facilitates the entire discovery stage of the workflow and, in some cases, elements of both the preparation and reporting stages as well.
7 Steps that Benefit from a Data Catalog
1. Search
An exploratory view of data allows people to do more than simply find the table they need; it empowers them to understand the larger context by providing a insight into all assets that speak to the larger business question. For this reason, the leading data catalogs center the user experience around the search function. Using simple, natural language search, an analyst can surface dozens of relevant data assets. Modern data catalogs, such as Alation, use machine learning to improve search results.
Like Google’s web search, these catalog search tools can catch typos, recognize synonyms, and use algorithms to order results by predicted usefulness. Those algorithms draw on metadata, or data about the data, that the catalog scrapes from source systems, along with behavioral metadata, which the catalog gathers based on human data usage.
2. Profile
Once the data catalog has returned several potentially helpful assets to a user’s query, the catalog also provides the analyst with the means to evaluate them. Most catalogs use a profile system not unlike an Amazon product page, which aggregates a variety of metadata about a given asset.
These profiles include basic statistics about the asset, like the number of rows and columns or the percentage of null values. They also contain more human-oriented information, including descriptions, ratings, and reviews by other users. Leading catalogs often have conversation features, where analysts can pose questions about the asset to its data steward or owner.
With these heuristics, analysts can determine if a data asset is actually relevant to the question they’re trying to answer.
3. Sample
This step is fairly simple. Most catalogs give analysts the ability to actually preview a small slice of the data from within the profile. From that sample, analysts can glean a better sense of the state of the data, how it’s structured, and how they might work with it.
4. Validate
Beyond sampling, analysts must take care to validate data in other ways. Many data catalogs provide data quality information, either natively or through partnership with a dedicated data quality tool. These tools use rules to validate the data and provide scores across a number of metrics, including whether or not the data seems accurate based on historic norms for that table.
Understanding lineage is another means of validating data. Analysts can also utilize the data lineage features of many data catalogs to confirm that the data asset in question came from the appropriate source and passed through the correct systems and checks.
Finally, crowdsourced feedback in the data catalog can greenlight good data – or warn others to proceed with caution. Users in a data catalog should be able to endorse particular tables as gold standards, or conversely flag other assets as deprecated versions. This establishes a shared source of truth for all analysts.
5. Annotate
Because analysts work intimately with the data, they often have unique insights into it. Catalogs offer features like comments and ratings, which allow analysts to share that knowledge with one another. This documents valuable tribal knowledge about data sources, saving time for other analysts who might not be as familiar with a given asset.
6. Query
Modern data catalogs have begun to branch out beyond their traditional domain of the discovery phase. Increasingly, catalogs also facilitate access to the data. They contain intelligent SQL query editors, which analysts can link to directly from asset profiles, meaning they no longer need to move to a separate tool to obtain the data once they’ve located it.
7. Promote
Lastly, some data catalogs even assist with reporting back the final answer to the business question. In the last few years, a few catalogs have begun supporting a data asset type that functions like a wiki for organizing the entire analytical process. Within one of these pages, a business user can ask a question and the analyst can respond, identify requirements, and ultimately provide the answer. This feature then makes that question-and-answer pair discoverable, so future analysts don’t have to repeat the same process down the line.
Closing Thoughts
Data analysts spend the majority of their time just hunting for, gathering, and validating data. A platform that helps them find data and understand it quickly enables them to spend more time, instead, on intellectually stimulating tasks–improving job satisfaction. At the same time, analysts’ increased productivity allows businesspeople to ask more questions and wait less time for answers. A data catalog provides critical functions to that end, especially during the discovery stage of the analyst workflow, but increasingly during other phases as well.
Learn more about how a data catalog can accelerate analytical insights. Download the white paper today.
- The Data Analyst Workflow
- 7 Steps that Benefit from a Data Catalog
- Closing Thoughts