Data Intelligence and the Scientific Method
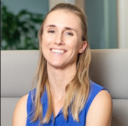
By Talo Thomson
Published on July 8, 2021
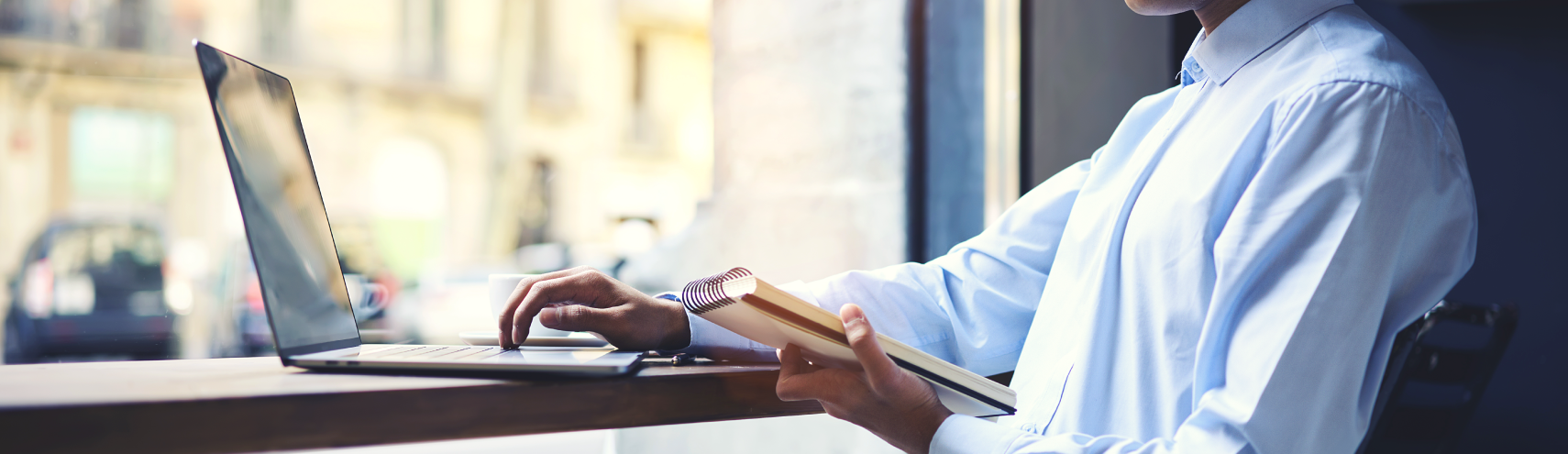
Got data? Odds are yes, and you’re lost in the trees of it! A central challenge for modern organizations is no longer gathering data — it’s navigating a veritable forest of it. Your average data analyst will spend three to six weeks just searching for an accurate, trustworthy dataset before they can get around to doing their job: analyzing, offering insights, and enabling others to see the data forest and the trees.
Data presents an opportunity. Data-driven businesses know this. They ask: How can we ensure we’re using data to grow smarter?
It takes a system. In his presentation, MIT CDOIQ: The Data Catalog as a Platform for Intelligence, Satyen Sangani, CEO of Alation, shares how that system inspired the structure of the modern data catalog. In this talk, Sangani details how academia offers the ideal model for growing data intelligence alongside human intelligence.
A Mess of Data: The Data Intelligence Challenge
Long before Satyen Sangani co-founded Alation, the first data catalog, he was a software developer at Oracle, which today is the second largest software company in the world.
“At Oracle I delivered and built analytical software,” Sangani recalls. “And through that process, I’d often end up seeing how much of a mess of data there was within our companies.” But that crushing volume of data wasn’t the real problem. “More fundamentally,” says Sangani, “ how people didn’t really know how to use it.”
When faced with a mountain of data, where does an analyst even begin? This is where Sangani’s background in academia came in handy (he holds his Bachelors in Economics from Columbia University and his Masters of Science in Economics for Development from the University of Oxford.) Academia boasts an established system for creating truth in the scientific method.
Truth as an Evolutionary Process
“I was trained in economics,” Sangani explains. “And you get this in academia, where there are lots of different papers, which become published, and offered to multiple different journals, and all of those papers cite previous articles from those journals, so you come to see this idea of truth as this evolutionary thing.”
Transparency is key. Academics use the database JSTOR (“Journal Database”) to research and cite from a canon of articles, which help them build more complex arguments toward a more highly evolved truth.
This got Sangani thinking, “from a software perspective, borrowing this academic model, how do you get to this intelligence?” He soon came up with a series of key parameters for data intelligence.
Data Intelligence and the Scientific Method Share Key Traits
You need the right information at the right time
You’re able to link to evidence (and show your work)
You have open access to all the data (to the extent that it’s legal)
You’re able to test multiple scenarios
You can access and add documented prose of the claim, the “truth”
The claims are traceable as they build on one another — both the datasets and the assumptions guiding them
These parameters are nothing new, as they’ve guided academics’ process — and progress — for decades. But in the world of software, applying this thinking was a breakthrough.
Inspired by academia, Sangani modeled the Alation data management framework on the scientific method: This means analysts have insight into not only who is using what data, but how they’re using it and the conclusions drawn. They have complete transparency into the supporting queries for a given report.
“Historically, there’s this idea, which many of us have heard about, which is called ‘the single source of truth,’” says Sangani. But his training in academia led him to realize that, when it comes to data management, the real need isn’t laying bare a “single truth.” What analysts and data managers truly need — just like academics — is a single system of reference.
“In this quest for intelligence, particularly in a world of physical information where evidence is distributed, what you really need is a single system of reference,” he elaborates. At Oracle, faced with that mountain of data, Sangani wondered: What if data analysts had a point of reference they could trust, just as academics have scholarly journals, the library, and JSTOR?
“Truth isn’t singular,” Sangani points out. “It’s this thing that exists in a lot of different places, and it largely depends on your point of view.” But a common source of reference enables data users to draw distinct conclusions from the same evidence — which all can trust.
“Your truth exists in systems,” he explains. “So there can be a truth according to the procurement department, which is different from the truth according to the finance department… and there may be a truth in the sales department that’s totally different! And every one of these systems is on some level a mask for a perspective on truth.”
Truth has many faces — even in the world of data. This makes a system of reference, paired with a process for truth-seeking, absolutely essential.
Conclusion
For an organization to grow smarter, data alone is never enough. Progress needs a process. Why reinvent the wheel? The scientific method offers a brilliant action model for hypothesizing, testing, and incrementally proving new truths. Academia is the shared point of reference for these agreed-upon truths. Alation’s framework for data management, modeled on these systems, enables data users to collaborate toward a fluid, evolving truth in a changing world.
- A Mess of Data: The Data Intelligence Challenge
- Truth as an Evolutionary Process
- Conclusion