Building a Data Governance Strategy in 7 Steps
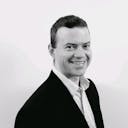
By Aaron Bradshaw
Published on December 16, 2021
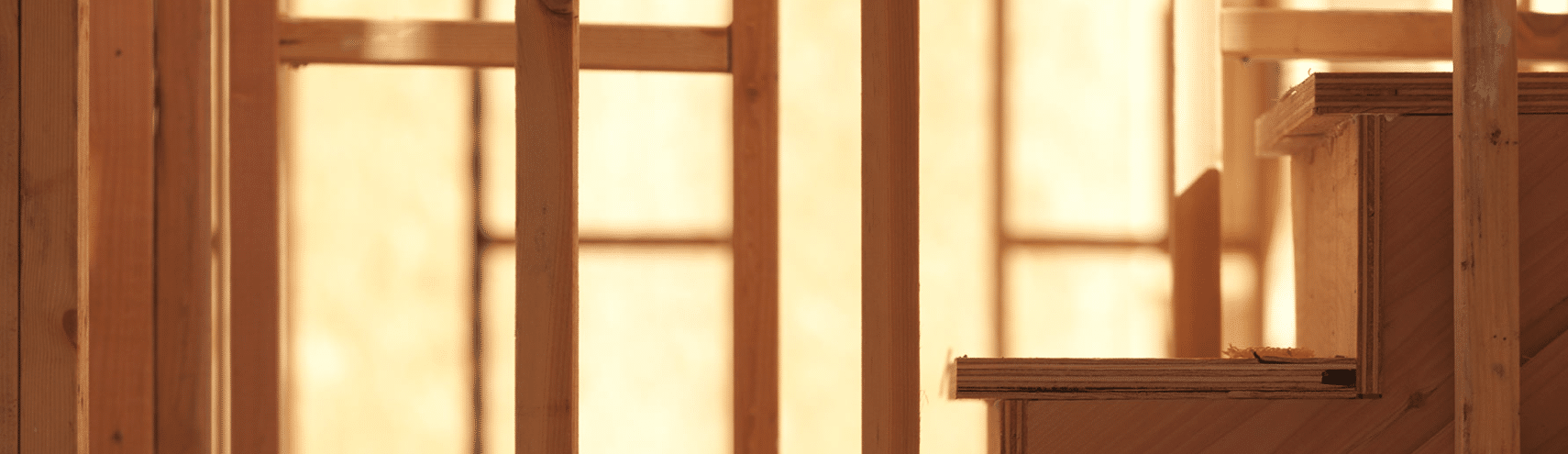
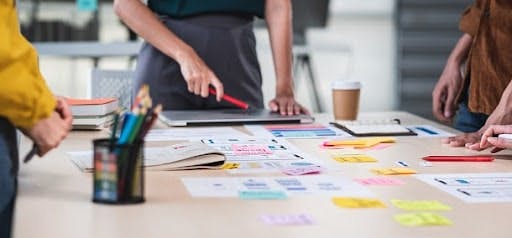
Modern business is built on a foundation of trusted data. Yet high-volume collection makes keeping that foundation sound a challenge, as the amount of data collected by businesses is greater than ever before. An effective data governance strategy is critical for unlocking the full benefits of this information. Advantages include more data-driven decision-making, enhanced business innovation, and improved collaboration.
Data governance requires a system. It’s important to have a solid framework of the people, processes, and technologies involved. This uncovers actionable intelligence, maintains compliance with regulations, and mitigates risks. Let’s explore the key steps for building an effective data governance strategy.
What is a Data Governance Strategy?
Data governance focuses on the daily tasks that keep information usable, understandable, and protected. A data governance strategy consists of the background planning work that sets the holistic requirements for how an organization will manage data consistently. This includes:
Assigning responsibility for implementing the policies and processes,
Defining policies for sharing and processing data,
Creating processes for naming and storing data,
Establishing measurements for keeping data clean and usable.
A data governance strategy provides a framework that connects people to processes and technology. It assigns responsibilities, and makes specific folks accountable for specific data domains. . It creates the standards, processes, and documentation structures for how the organization will collect and manage data. This ensures integrity by keeping data clean, accurate, and usable. Through this foundation, you ensure secure data storage and access.
Why is a Data Governance Strategy Needed?
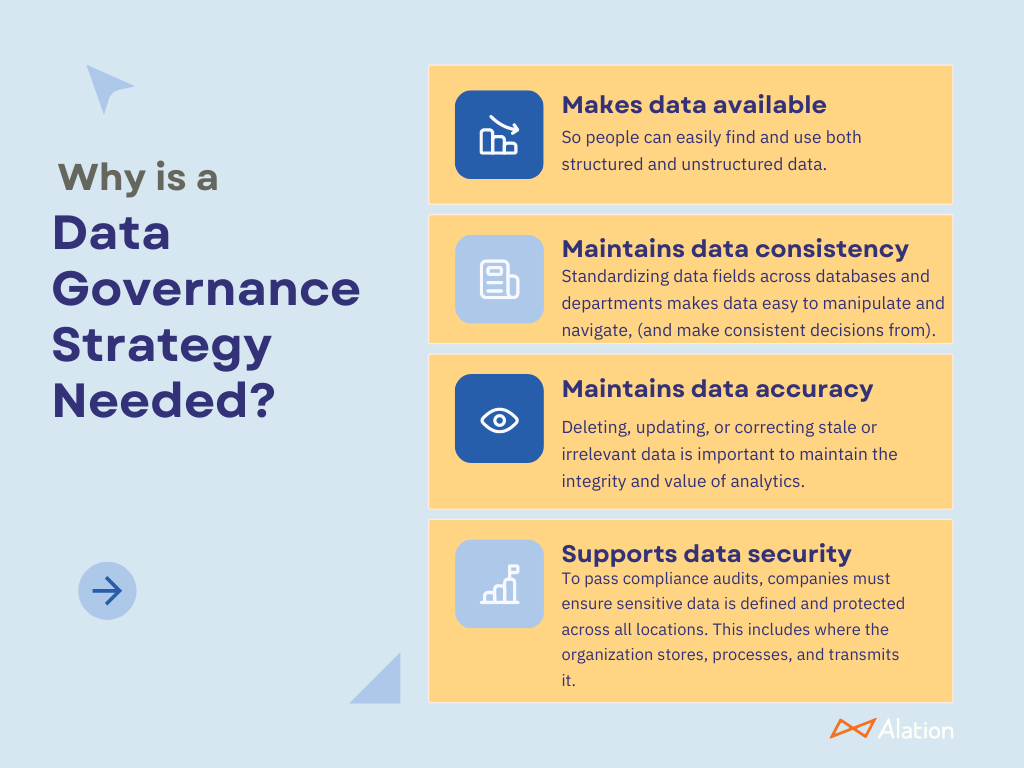
There’s an old saying that “bad facts make bad law.” And in the world of analytics, bad data makes for bad decisions. A data governance strategy helps prevent your organization from having “bad data” — and the poor decisions that may result!
Here’s why organizations need a governance strategy:
Makes data available: So people can easily find and use both structured and unstructured data.
Maintains data consistency: Standardizing data fields across databases and departments makes data easy to manipulate and navigate, (and make consistent decisions from).
Maintains data accuracy: Deleting, updating, or correcting stale or irrelevant data is important to maintain the integrity and value of analytics.
Supports data security: To pass compliance audits, companies must ensure sensitive data is defined and protected across all locations. This includes where the organization stores, processes, and transmits it (details an organization must be ready to share with auditors, or increasingly, with individuals whose personal data has been captured and seek to have a say in how companies use it.)
A data governance strategy helps organizations gain greater value from data science and business intelligence tools, as well as the analysts and scientists utilising them. At the same time, it enhances data security and compliance programs.
How Does Data Governance Support a Data Strategy?

A company’s data strategy focuses on all people, processes and technology required to effectively make use of data assets to generate business value in line with the business strategy. This could include operational efficiency, minimising expenditure, risk mitigation, or revenue increase.
Data governance sets out the foundation for organizing data and its related assets, and establishes policies and practices for keeping data secure and usable.
A data governance strategy is a subset of the wider data strategy; it creates the framework to govern data effectively, and allows the wider data strategy to be met and delivered upon in line with the business strategy.
Defensive vs Offensive
Defensive data strategy focuses on minimizing risk. The activities associated with this include:
Regulatory compliance mandates like data privacy and financial reporting laws
Detecting and mitigating risk of fraud and theft
Identifying, standardizing, and governing authoritative data sources
On the other hand, an offensive data strategy supports business objectives. These activities include:
Gaining insight about customer needs
Integrating customer and market data for planning future business goals
Supporting the sales and marketing pipelines
Operational efficiency and process improvement
Organizations need to balance offense and defense, and a robust data governance strategy can help. For example, governed, labeled data makes it easier to put appropriate privacy controls over sensitive information and ensures more accurate analytics, as long as this information is centrally located and well-curated, EG, ready to be consumed by all.
Enabling wider access is important, as silos create inefficiencies. Governing your data in a tool only viewed by governance and privacy teams misses out on the large community of analysts and scientists struggling to find, understand, trust, and use data in innovative ways.
How to Develop a Data Governance Strategy
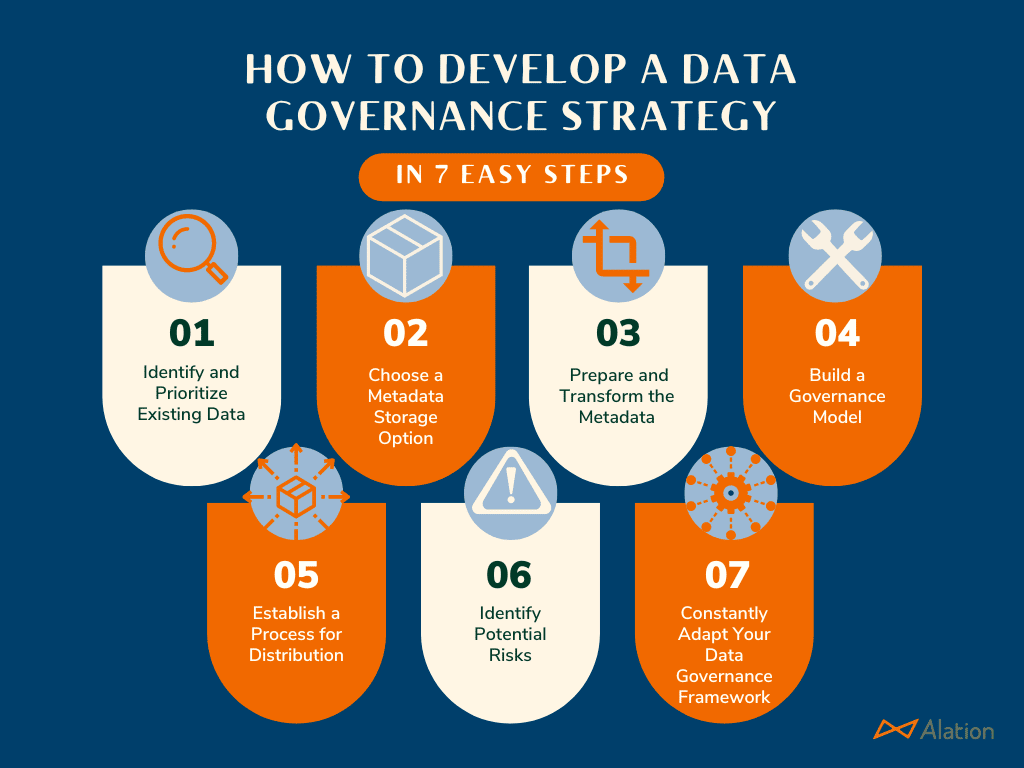
What does the business seek to do with data? Your data strategy should be clear and easy to communicate in simple language. If only a data scientist can understand the strategy, it’s unlikely that strategy will be successful, if everyone is to get onboard. Governance plays a key role in supporting that strategy at every step.
Where does your business sit? For companies in highly regulated industries, like financial services or healthcare, data strategies are most often compliance focused: defensive. They may set a data strategy focused on protecting private health data and passing compliance audits. In this case, governance ensures key processes are documented for future audits. Increasingly, even across these industries, the CDO’s success is determined by the positive value delivered. CDOs are increasingly de-prioritizing defensive strategies as a result.
By contrast, less regulated industries, like retail, are freer to pursue more aggressive, offense-focused strategies. An example of an offensive data strategy may be to use data to drive sales in a new location, or to gather competitive intelligence for a new product launch. In these cases, governance will structure processes: how insights are gleaned and key choices made.
Conversely, the rise of personal data regulations is causing these industries to re-prioritize defense. These industries are having to increase their scope to cover their defensive needs, in addition to the value-add initiatives that support a competitive edge.
But before governance can support a strategy, data governance must be implemented. Let’s take a look at the seven key steps for implementing data governance:
1. Identify and Prioritize Existing Data
To implement a data governance strategy, a company needs to know what data it already has. As part of this process, the organization should start by:
Inventorying data: Create a complete record of information resources with relevant metadata
Classifying data: Analyze structured and unstructured data to organize it by relevant categories
Curating data and knowledge: Organize and manage datasets with active metadata management and data catalogs
2. Choose a Metadata Storage Option
Traditionally, departments within a business have their own databases for metadata management. This has led to siloed data which limits the sharing and reusing of metadata assets. Choosing a storage option that centralizes metadata is key for:
Collection across many platforms
Productive reuse of metadata
Visibility into data history
Effective governance and stewardship
Centralized metadata ensures scalability and flexibility needed for analytics. It also helps different departments understand the value of data lineage.
3. Prepare and Transform the Metadata
This is one of the most time-consuming steps. It requires going back to the raw metadata, reformatting it, correcting it, and combining datasets into data catalogs. The three primary activities are:
Cleansing and validating data: Removing outliers, filling in missing values, standardizing the data, masking sensitive entries
Transforming: Updating values or formats so that all data can be understood and used across the organization
Creating templates: Create templates for a business glossary, data dictionary, and business metadata. This will help organize data vocab and track how many data assets or terms you upload within your network
4. Build a Governance Model
A one-size-fits-all data governance model does not exist. Historically, companies used passive, compliance-oriented frameworks. These defined how users created, stored, maintained, and disposed of data. However, to take advantage of all that analytics offer, companies need modern data governance models that:
Respond to multiple styles and are sensitive to contexts
Encourage innovation
Provide a flexible, dynamic strategy across enterprise and ecosystem
Incorporate distributed decisions rights connected to value
Take an active approach to managing risk proactively
The governance model must also be centralized or federated. And the model you choose should be dependent upon the needs of your organization:
Centralized Governance Model: A centralized governance model is when one group determines the rules of how to govern data. This group defines critical data elements and how to approve business terms around data. It also addresses core processes that every team member must adhere to.
Federated Governance Model: A federated governance model is when several groups have authority over data. This is helpful when departments have different data needs.
5. Establish a Process for Distribution
Modern data governance strategies should democratize data. Data governance policies only work when people follow them. This is why policies are most effective when they are embedded into people’s normal activities, workflows, and tools.
To mitigate regulatory risk effectively, organizations should consider:
Appropriate employee onboarding
Training employees around usage policies and guidelines
Encouraging knowledge sharing among employees
Creating processes for requesting and making changes
6. Identify Potential Risks
New security laws and compliance requirements are continuously emerging. For example, the California Privacy Rights Act (CPRA) and the General Data Protection Regulation (GDPR) require companies to put appropriate security controls in place.
Potential risks that companies need to account for in their data governance strategy include:
Excess access: everyone should have the least amount of access necessary, even at the data field level, to do their job
Secure storage locations: all sensitive data storage locations need to have security controls in place to prevent cybercriminals from accessing or stealing data
7. Constantly Adapt Your Data Governance Framework
Businesses change, and so too their data strategies. Companies need to continuously adapt and improve their data governance processes. This allows them to respond to issues like rising data privacy risks as they emerge.
Organizations need automation that helps track and measure the effectiveness of their strategies to:
Determine policy conformance
Measure data usage
Enforce consistent data quality
Analyze curation
Throughout the Process: Facilitate a People-Centric Approach
A key element of the data governance process is to focus on the people. The goal is to create a data culture that encourages people to take responsibility for assets in the data catalog.
When you implement a data catalog, and ingest new assets, it’s vital you identify your stewards, or those who know the data best. Those who use an asset the most are your subject matter experts on that asset. The Alation Data Catalog auto-assigns stewardship based on actual, individual usage.
Then, it’s important to encourage your stewards to share their tribal knowledge by rewarding them with recognitions, such as certification levels, badging, etc. Other forms of recognition include monetary bonuses, physical gifts, and extra time-off.
This approach gets users excited to adopt ownership. It also gives them confidence that their knowledge source can be undeniably trusted. In turn, this empowers users to more effectively leverage the information in front of them.
Final Thoughts
Data governance can play a key role in supporting your data strategy. But earning the support of your community is crucial. From an organizational point of view, people need to be involved in understanding how their departments use information and why they need access to it. At the same time, these data stewards should have automation, including machine learning and crowdsourcing capabilities, that help them maintain these processes.
Organizations are generating more data than ever. Data citizens need data governance solutions that help them continuously enforce and improve processes. Setting benchmarks is crucial for improving processes, which is why monitoring and measurement tools are essential. These tools allow people to track data curation against policies and standards.
The core of a strong data governance program lies in getting people to take accountability. To do this, companies need to develop strategies that are most likely to be adopted and operationalized across teams.
Alation takes a people-first approach to data governance. This means companies can organically launch governance based on what people are already doing. Once a non-invasive framework is set up, companies build an iterative process of continuous improvement without obstructing day-to-day work. This aligns people, process, and technology — with people leading the charge. Simply put, Alation empowers a community of willing participants to learn from subject matter experts, who share their expertise on the platform.
- What is a Data Governance Strategy?
- Why is a Data Governance Strategy Needed?
- How Does Data Governance Support a Data Strategy?
- How to Develop a Data Governance Strategy
- Final Thoughts
Contents
FAQs
A data governance strategy consists of the background planning work that sets the holistic requirements for how an organization will manage data consistently.
A data governance strategy helps organizations gain greater value from data science and business intelligence tools, as well as the analysts and scientists utilizing them.
A data governance strategy is a subset of the wider data strategy; it creates the framework to govern data effectively, and allows the wider data strategy to be met and delivered upon in line with the business strategy.