Competing Apart, Sharing Together
with Michael James, SVP, Head of Data Strategy and Analytics at the NBA
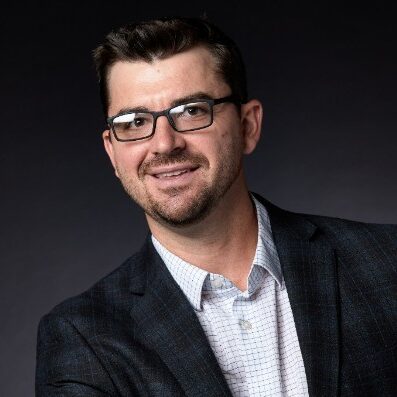
Michael James
SVP, Head of Data Strategy and Analytics at the NBA
As the head of data strategy and analytics at the world’s premier professional basketball league, Mike James leads the NBA's centralized business strategy and analytics efforts. Before joining the NBA, he worked in labor finance at the NFL, and graduated with an economics degree from Harvard University.
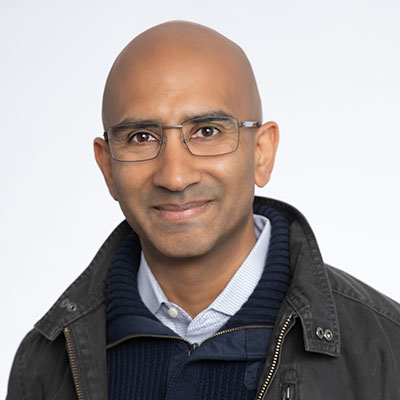
Satyen Sangani
Co-founder & CEO of Alation
As the Co-founder and CEO of Alation, Satyen lives his passion of empowering a curious and rational world by fundamentally improving the way data consumers, creators, and stewards find, understand, and trust data. Industry insiders call him a visionary entrepreneur. Those who meet him call him warm and down-to-earth. His kids call him “Dad.”
Producer: (00:00)
Hello and welcome to Data Radicals. In today's episode, Satyen sits down with Mike James of the NBA. Mike is responsible for the league’s centralized business strategy and analytics function, and oversees business intelligence and global data strategy. Much like the NBA's commitment to diversity, Mike is on a mission to broaden the pool of people interested in the data field. In this episode, Mike and Satyen discussed the NBA's digital transformation, the future of GenAI in the league, and attracting more people to sports business analytics. This podcast is brought to you by Alation. We bring relief to a world of garbage in, garbage out with enterprise data solutions that deliver intelligence in, intelligence out. Learn how we fuel success in self-service analytics, data governance, and cloud data migration at alation.com.
Satyen Sangani: (0:58)
Today on Data Radicals we have Mike James. Michael is the SVP and head of data strategy and analytics at the NBA. In this role, Michael connects executive leadership, technical expertise, and embedded business analysts to create a data-driven approach to decision-making and to support the league's constant pursuit of innovation. Prior to joining the NBA in 2011, Michael worked for the NFL in labor finance, where he focused on the collective bargaining agreement. He also holds a degree from Harvard University in economics. Michael, welcome to Data Radicals.
Michael James: (01:31)
It's great to be here.
Satyen Sangani: (1:31)
So, tell us a little bit about this role of being SVP of data in a sports league. What does that mean and why does that job exist and what are the analytical needs inside of a league like the NBA?
Michael James: (01:42)
This job really has come about over the past decade or two. This wasn't always a historical function that existed within sports, but through the years, sports, much like any other industries, has really realized the power that data can have in helping us at its core engage our fan in new and different ways. When you think about my day-to-day and what I do here at the NBA, it's very much about helping the NBA use data to make better decisions. That's the core of this role: making sure that we are able to make better decisions, leveraging data that can manifest itself in a variety of different ways as part of the day-to-day. But that's the fundamental function of this role. And it's something that, again, we've seen tremendous growth at leagues, across teams, and shoulder industries and sports in this data function over the past decade or two.
The genesis of D&A in CRM
Satyen Sangani: (2:43)
And tell us about some of the data problems that exist. Any sports fan, particularly in the major sports leagues, sees players and sees all of the advanced analytics. In basketball, one of the more recent or relatively more recent ones is plus/minus. And you think about sort of analytics from the perspective of understanding players and their relative contribution. Is that where your time and energy are focused or are you focused on something else? And what are the use cases for analytics where you see the most demand for information?
Michael James: (03:15)
When you think about Moneyball and you think about the story that was brought to life there both in Moneyball by Michael Lewis as well as the Moneyball movie, that's what people think of when they think of analytics in sports. And I think it's kind of assumed that there is also similar work going on on the business side. But really that was where things started in sports was on the player side, leveraging data to be able to get an edge in either evaluating talent or helping to devise strategy and tactics for how to approach on-field, on-court play. But the business side took a little while to catch up and it's always a funny story when I get asked, “How long has there been data strategy analytics on the business side of these sports properties of teams?” And it actually is something that really came after the release of Moneyball in the advent of the player side data strategy and analytics function, which often catches people by surprise.
Michael James: (04:10)
This function started — its advent was on the ticketing side. That's where this function grew up. The idea that there is a more data-driven approach that could be taken in a very discreet fashion for how teams sell their tickets and how they price their tickets and who they look to to target their tickets to. And so that was really the key function that started it all. And if you go back 12 years to when I first joined the NBA, when we think about the data strategy and analytics function, usually it started with a CRM analyst, a CRM manager. That was the role that you tended to see most to sort of make the foray into this space was this idea that we need somebody that can gather up all the potential leads we have in the ticketing space. And then that person also kind of moonlighted as a pricing analyst and they helped you set the pricing for the tickets. But basically it was like, who can we target? How much should we sell the ticket for? From there, the industry has exploded, but that really was the roots. That was the initial use case that got this industry started in the data strategy and analytics space.
Dribbling through dense data
Satyen Sangani: (5:14)
And CRM is an interesting concept in the context of a sports league because your end customers are of course people who are buying tickets and the fans. And how do you maintain a relationship with those fans as a league? Do you work through the CRMs of the individual teams? Is that primarily season ticket holders? Customer intimacy is hard because some of it's via digital mechanisms and through all of these networks and some of it's through the actual ticket buyers and then people are buying merchandise. That's a fairly complicated thing. And also there's multiple levels of indirection between the networks and the actual teams. How do you navigate all of that data?
Michael James: (05:51)
It's a rapidly evolving space. As you see more and more leagues get involved on the direct-to-consumer front and these products that they're working to market actively directly to fans of the league as a whole. Our teams obviously have very specific use cases for how they want to engage their fans and what they want to use CRM and now data warehouses or customer data platforms, what they want to use all this technology for. It's really to focus on the in-arena experience, driving fans in-arena, making the in-arena experience as fantastic as we can make it, leveraging data to do so. So, those are use cases that the teams will have. Now, I would say we also, at the league, put on events. That's a key part of what we do as well. But at the league level, really what we're heavily focused on now is our next-gen app and our next-gen product.
Michael James: (06:50)
And that direct-to-consumer link through that product. And I'll talk a lot about this, but we're heavily use case focused. Data needs to have a purpose, there needs to be a decision that you're making. There needs to be a fan behavior that you're trying to affect. So, those are different between team and league, but they are all connected. And certainly we do a lot of work here at the league to link those team and league behaviors, to link the data that's driving the way a team wants to connect with a fan, and the way that the league wants to connect with that same fan. They're different, but related.
30 teams, 30 data approaches?
Satyen Sangani: (7:20)
In your role, you mentioned the use case focus and you also mentioned that the teams are driving their own relationships. Is the technology architecture for every given team highly different or is it fairly homogenous?
Michael James: (07:31)
It's an interesting way to think about it. I think “homogenous” in as much as the technology stacks contain the same types of products. But very heterogeneous, when you think about the different vendors, the different partners, and the different ways that teams go about solving these data-driven problems. It used to be that we would have a couple of different, say, data warehouse vendors playing in this space. And the differences you would see would be in terms of who a team decided to partner with, but the end product would be somewhat similar. Now, we see a variety of different approaches. We'll have teams that are out there building their own data infrastructure on their own internally. We'll have some teams that are partnering with a vendor in one way, partnering with a vendor in a different way. So now, all 30 of our NBA teams have a data warehouse, but what that means is entirely different.
Michael James: (08:32)
And how they get there is entirely different. We encourage that. That's part of our philosophy, is that we think of our 30 teams as individual laboratories for growth. Laboratories for experimentation across a lot of the business, especially in the tech space. And that we think helps teams keep their technology, their stack focused on the use cases that they wanna solve. That's not the same for every team. So we like this approach where teams get to go out and they get to build a stack that's right for them. And it will look different team to team, it will look different between the team and the league. But what we all have in common is we are still facilitating the links to keep this information flowing between all of us.
Satyen Sangani: (9:10)
So, there is a different warehouse and presumably even data model. There's obviously common artifacts like a season ticket holder, a season ticket holder, and a seat’s a seat and a vending machine’s a vending machine. But you're saying that for the Los Angeles Lakers and the New York Knicks, you've got two totally different technology stacks and possibly data models and data warehouses. And each of these teams is kind of doing it their own way as they choose. Is that right?
Michael James: (09:36)
Correct. Yeah. And again, quite simply, the reason for this is that every team is going to have a slightly different set of use cases to fill. One of the things I appreciate most about the growth in sports data strategy and analytics or business strategy and analytics is the number of different vendors, products, and solutions that are serving the space now. It used to be that we would have to take these behemoth solutions and whittle them down to work for specific sports use cases. And then you started getting players that came into this space that offered sort of one solution that was more tailored to sports, but it was still one solution. Now, there's so many different ways to solve data-driven problems and to build your technology stack to be able to facilitate doing so that we've really seen our teams take very different approaches based on the use cases and the problems that they're trying to solve.
Satyen Sangani: (10:29)
I find this to be super interesting with data analytic tools because unlike, for example, a finance system, you can book an accounting transaction only in so many different ways. That is not true about data and analytical problems; there are so many different ways of doing it. And I think what makes your circumstance in the NBA circumstance and maybe your role particularly interesting, is that you've got this Petri dish of 30 different teams or 30 different Petri dishes of teams who are kind of solving the same problems, but to your point, different ways. And you would think that given the similarity of the business models across the teams, that they would be doing it in the same way. Is there best practice that people are trying to share between the teams in order to be able to get to a place where people are learning from how the other ones have done it better or well or badly?
Michael James: (11:14)
There's tremendous infrastructure that has been built at the NBA — really over even spanning back more than two decades now through a group — called Team Marketing and Business Operations, or TMBO as we like to call it, internally. That's their sole focus is, how do we transmit best practices across the league. They'll do that in a variety of ways. Working very closely with our data strategy and analytics team. I'm a TMBO alum from a decade between 2011 and 2021, so I know this space well. But what they're heavily focused on is either through live workshops with our executives at teams, calls that we have on a cadence with our teams and other ongoing touchpoints. How can we share out “Team X did this great thing on the ticketing front, everyone should be doing this.” We have mechanisms to create those best practices to codify them, solidify them, share them out either via internal intranet platforms that we have set up to share best practices or through any of those tentpoles, calls, workshops.
Michael James: (12:20)
So, we're active in that space, incredibly active in that space because the philosophy of TMBO going back to its start was, we are going to compete heavily on the court. So all of that side, and in my role in data strategy and analytics, I oversee the business side. I do not oversee the player side and there is a separation there, on the player side, there is a lot less sharing that goes on between our teams because our teams want that competitive advantage. But on the business side, there is a lot more sharing that goes on between our teams because we want all businesses to do well and there are things that work in one market that might work in another market. And that's the fundamental philosophy of TMBO. And it's a mechanism to this day that makes it incredibly easy for stakeholders of TMBO, like our data strategy and analytics group and other entities throughout our league office to be able to leverage TMBO to be able to facilitate best practice sharing across our 30 teams.
Sharing the (data) ball among the league
Satyen Sangani: (13:16)
I just happened to run into this guy named Brandon Schneider, who I had never met before, but happens to be the president of the Golden State Warriors. And he literally made the exact point and I said, “Yeah, you know, I'm about to talk to Mike and we're gonna do this thing on the podcast.” And one of the things he said was, “That's super interesting because even though on the court, all of us are fighting with each other on the operations side of each basketball team in order to win the title, off the court, we're basically all of one brain because when the Atlanta Hawks make more money, we make more money on balance. We ought to be sharing these best practices.” And on some level what's interesting from the perspective of a lot of the listeners of this podcast, many of our customers, Alation's customers, and folks are trying to build data culture in multiple business units. And so this kind of sharing concept is super interesting because it puts you in the role — the central NBA organization — in the role of having to spread these best practices. Tell us a little bit about how you discovered those best practices. Like how do you know something works and how do you know something doesn't work and when do you start to share and once you've shared, how do you think about publicizing what's great and maybe even what doesn't work?
Michael James: (14:22)
This is, again, a practice that has been built up over a couple of decades now. But it starts with, we have very active communication with our teams. You build up a relationship over time and you start to realize if this person is sharing this thing that worked, we have a good sense of who else might be able to benefit from it. We hold these all-30 team calls, you get a sense of interest. So if someone shares something, you get a sense, oh, if they get 10 questions, this could be something that we need to dig into further. And then we also have an incredible amount of expertise at the league as well. It's pretty easy for the subject matter experts to dig in on a particular example and say, they've got something here, we've gotta get this shared out. And again, at the upcoming touchpoint, be it a monthly call, be it a workshop, we'll make sure to package that up in a way that is not only informative — “Here's what the team did” — but also has the tangible next steps.
Michael James: (15:19)
“Here's what you can actually do with this to try the business.” And it's no different on the data side. We've built a ton of data products through the years at the league level for our teams, also for different departments within our league office as well. The goal of all of those products is to make sure that we are driving better business decisions, we're driving a better fan experience, and ultimately that's gonna lead to more revenue. When we roll out a model — about 10 years ago, we rolled out our first edition of our lead scoring model, which we called FICO. It's a different acronym than the FICO that you may be familiar with. But basically what it was was an expected value model for our fans based on activities they've undertaken so that our teams and the precious commodity which they have, which is time for our sales rep, could be talking to the right prospects to be able to sell a potential season ticket membership to, or a partial plan to.
Michael James: (16:17)
And this was something that we built in about the 2011, 2012 timeframe, but it took a series of years. Let's have, let's work with this team really closely on how to roll out this model. Let's see how they roll it out to their sales reps. How do they allocate the leads across the sales reps? Okay, great. Now that we've gotten that, we're gonna go ahead and package that up. We're gonna share that at the next workshop. Okay, now we've got five more teams that are incredibly interested in doing this. Let's work with them. Let's read out again. And that's the approach we took for every product that came after that. Shortly after we released our season ticket member retention model, which took a look at all of our season ticket members across the league and what could we learn about their propensity to renew.
Michael James: (17:00)
And that helped our account reps at our teams be able to understand who are their fence sitters, who are the folks that are very likely to renew, what's the strategy across each of these cohorts and how to approach? And again, we didn't start with all 30 teams there. You start with a few teams that are interested and you start to build out, here's what worked, here's what didn't, let's take the next 10, see if you can tell us what works and what doesn't. And then ultimately you get to the entire league. So there's always that, that stepwise approach. And it's something again that TMBO has really gotten down to a science through the years.
Establishing best practices across an entire enterprise — or league
Satyen Sangani: (17:34)
It’s sounds super fascinating and it's pretty instructive for any data analytics leader. Because you might think that, or at least one would suspect that in your role you might have sort of horizontal data across all 30 of these teams. And so maybe you're just generating insights and like saying, “Hey, I have this insight today and this insight tomorrow.” Sounds like what you're saying is, “No actually we just go deep with one team, try to actually incorporate the data into their business process, learn from that, and then scale it out based upon the actual business impact.” Is that a fair summary of what you just said or did I miss it?
Michael James: (18:03)
Very fair. I think the one add I might make is there are some products that are self-evident and scalable such that we have this platform both for our teams and a separate one at the league called TMBO reports and NBA reports, respectively. And this is just a set of reporting suites, dashboards, insights, newsletters — a variety of different things comprise these reporting suites. But they are intranet sites that are accessible to all of our teams and to the league office, respectively. For some of those it's pretty patently obvious what the reporting is for, how it can be used, what you can get out of it. For others, it's a little bit more complex, it requires a lot more hand holding. So I think what you have to do as a data leader is really understand what are the stakeholders gonna get, take, run with.
Michael James: (18:50)
They don't need a lot of hand-holding. And what are the things that are gonna take a little bit more convincing? What are the things they're gonna take a little bit more of the evidence building to get adopted? Those are where you dig in a little bit more, you approach that more on the one-to-one basis. Sometimes a group of five or 10 that we'll start with doesn't have to always be one, but it's certainly not something where you try to go all 30 at the beginning and then you kinda see, “It didn't really take anywhere.” You get a sense which products on a product by product basis are gonna be the ones that you can kind of let the market place run with, and what are the ones where you're really gonna have to spend your time and make sure that you win stakeholders.
What’s working in D&A?
Satyen Sangani: (19:27)
You, in dealing with these 30 different teams, probably observe 30 different levels of data culture at these organizations, and there's, I would imagine gotta be leaders and laggards and people who are doing it, maybe there's not one that comes up with all the innovation, but certainly there's gotta be a distribution. What can we learn from the habits and the behaviors of the ones that are doing it better, or do they invest more, or what are the things that sort of stick out to you as differentiators between the ones who are just better at data analytics?
Michael James: (20:01)
I think it really all comes down to the influence on business decisions, and I go back to one of my favorite debates in the space — again, outside of my wheelhouse — but it's a debate I think we've all seen, which is you'll get basketball pundits together and they'll talk about analytics or gut when it comes to “Should it just be no numbers or should it be all numbers,” and I just shake my head every time I see that argument because I'm like that kind of displays a fundamental misunderstanding of how data and analytics are supposed to work. Data and analytics works best when business leaders who are about to make very consequential business decisions have been highly trained in how to create hypotheses that are testable and then can give those hypotheses to people who are trained in leveraging information.
Michael James: (20:52)
I don't even wanna use the word “data” here, but information and evidence to test those hypothese. That's the inner play that data leaders are looking for and that business leaders are looking for between the two areas. So, I think that to that initial debate, it really is the folks who have all of the experience and that gut intuition should be the ones generating hypotheses and the analyst, the analytics folks, should be the one testing those hypotheses. So to get back to your initial question, the organizations that are doing the best are the ones where the business leaders are forming testable hypotheses with data and want the data and the information and the facts to lead them to the best decision they can possibly make. Not the perfect decision, not 100% likely to be right. Our role is really to be more card-counter than decisive answer. We wanna tilt the tables a little bit in our favor and make better decisions. We’re never gonna make perfect decisions, but the interplay between the business leader that's making that consequential decision and the data and analytics leader, if that's really strong and they have that: “I think this. What do you see? Okay, great.”
Michael James: (22:04)
“You see this. Yeah, but did you look at it this way? Okay, great. You looked at it this way.” I think we have the best decision we can make, there are gonna be the organizations that are gonna grow their data and analytics function because they're getting stuff out of it, and they're gonna be the ones that see the most success with it. So, generally, that's the common through line you see in organizations from a data perspective that grow, that have all the technology they need and have the resourcing that can help support what they're trying to do.
Maximizing data engagement
Satyen Sangani: (22:32)
Yeah, you're in good company. We had a podcast with General Stanley McChrystal, who broadly said the same thing. He's like, “Look, you've got a soldier on the field and you've got these guys who are running around and killing terrorists, and they've got biceps that are bigger than most people's chests and they're poring over, looking at data, and they're trying to understand it so they can make a decision in a very subjective field of war.” How trainable is that and how much do you guys invest in training? If that's true, then you would expect that a big part of building this sort of culture of data is about teaching people to make decisions or think about how to make decisions. Is that work that you've either observed or seen happen or have any insight into?
Michael James: (23:15)
I would say that the approach that we take when it comes to helping stakeholders, for lack of a better term, is to figure out how to interact with our group and figure out how to react with data. Really, it's a two-part engagement. Part one is, What are the questions you have about your business that you've always wanted to know? Maybe there's some of them that we can help you answer. And what stories are you looking to tell? I always refer to this as this form of stakeholder management is kind of like if you're on an icy road and you're driving around and your car starts getting out of control, the instinct is to grab the wheel and turn it right back on the road.
Michael James: (23:57)
But we all know, I grew up in the South, so I wasn't aware of what you do on icy roads, but now I've learned that you steer into the skid first, and then once you have the car under control, then you're able to gradually turn yourself back on course and that's sort of how I view stakeholder management, the space for those that don't have a good sense of how to interact with data to drive decisions. First, you start figuring out what is the stakeholder's problem set. What are they trying to solve? What decisions are they trying to make, and what do they wanna know, and working incredibly diligently to rack up wins there and to make sure that you're solving their problems first. Because there's a level of trust that needs to be built up and so working as hard as you can to give them wins from data, and then you start to say, “Okay, how about if we looked at it this way?
Michael James: (24:43)
“What if when you make a decision, you think about these three things, and then we go out and try to figure out the answers?” You start inserting those like, here's the way that we might approach this problem. Still doing what the stakeholder needs, but slowly over time, injecting that sense of “We could probably get you a better answer if you looked at it this way.” That's our approach, we obviously, at the lead, we have a ton of ongoing management training that goes on across disciplines. So, there's certainly the ability as well for our mid-level leaders all the way up to our senior leaders to be able to take ongoing education courses, including in the data space, but I find that the best learning comes in that direct communication and in that very practical — to borrow from General Stanley McChrystal — on-the-battlefield training, where you're really in a live situation and you're able to sort of work with that stakeholder and share with them the power of this can have.
Empowering your stakeholders
Satyen Sangani: (25:44)
That strikes me, as you think about this sort of process of dealing with the stakeholder and in some sense asking them, “Hey, what questions do you have of data?” That is an open-ended question is that... Are you formal about that? Are you actually having meetings where you're like, "Look, we're gonna have a quarterly meeting where we would love to know what things we'd love to know about the business, and we're actually being that open-ended about the process." Or is it just in the work you're just having conversations and all of a sudden questions come? How formalized is that interrogation of discovering these key business questions that people are working to answer to.
Michael James: (26:18)
Generally, it happens in a few different ways. Sometimes it'll happen in discussions with the different departments that we serve across the league, also with our teams, and I should also mention our regional offices as well. It's usually less about “What could we use data to help you with?” and it's a little more around “What are the business challenges that you're facing? What are the questions you have about your business?” Sometimes those are self-evident, sometimes that's the reason for the meeting. It's a little bit easier to understand what we're gonna be talking about, and what we're gonna be focused on in those situations quite frequently will be a little more proactive with, here's the data we have that can help you solve this problem.
Michael James: (26:56)
But other times, it is a less formal check-in where it's more about, broadly, what are your business objectives and what are some ways in which we can leverage the data asset that we've built here to be able to support you, and it's not even always about data — sometimes it's just about the capabilities. Sometimes it's someone who just wants an answer to a question that may involve a lot of work in a different tool or something that our group can bring to bear. It's not even always necessarily explicitly a data question. We're just here to help make better business decisions and help our stakeholders in that endeavor. So there's some of that. Then we also have an executive committee of leaders throughout the organization, a cross-functional group that essentially is loosely the board that oversees this general data project we had initially invested as a league in our customer data strategy project, what we called at the time, in 2016.
Michael James: (27:46)
And ever since then, we've had this notion of a steering committee that is going to be able to help the project be able to understand the strategic objectives of the organization and make sure that the project was aligned with that. We’ve since morphed what was our customer data strategy project into this broader data strategy and analytics focus, and along the way have developed an incredible focus and push behind our next-gen product and developing D2C capabilities here at the league.
Michael James: (28:21)
So, while the focus has changed a bit, that notion of needing a group of executive stakeholders to help keep our group focused on the right strategic objectives for the organization has endured and they're an invaluable resource for us to be able to understand, “Okay, here are the key objectives of the organization, here are the areas where we're definitely gonna need how when it comes to making very important strategic decisions for the organization.” So, either get data ready to support or we're looking for these three different areas of exploration, but that group is invaluable both to helping our groups stay focused on what the organization is focused on, as well as to ensure that buy-in across the organization, because it's such a cross-functional group that everyone has awareness into what we're working on.
Cultural differences within the league
Satyen Sangani: (29:09)
Yeah, it's funny, as I think about some of the data practices that we wanna implement into building a data culture, we talk a lot about our business problems and we obviously talk a lot about what we are trying to do, but we don't necessarily create that open space to sort of say, “Hey, what are the questions that we would love to be able to know?” And I think starting meetings, or at least some meetings where that open-ended prompt could be really great to both train people to be curious, but also to discover what really matters within the organization. So it's a super, super interesting practice as a general idea. Switching gears a bit, you obviously now have seen 30 different teams, you've also seen two different leagues. And I'd love to understand from you what are the differences in culture and how do they differ, and where do you see as being similarities?
Michael James: (29:55)
I have found that across sports broadly, there has been a tremendous increase in, I guess what you could broadly define as a data-driven culture, but a desire for building out a data asset that can help solve business problems. And I think when you look back to when I first joined TMBO at the NBA within our league, we did a survey, we asked, “How many folks do you have within your team that are fully dedicated to strategy and analytics?” And we got the survey back, and it was 22 people, and we do this every year now. But you look a decade later, that had gone up more than tenfold. We’re at the point now where our average strategy and analytics group across our league is right at about 10, which is just remarkable. Consider that this was a sales and marketing vertical that almost didn't exist a little more than a decade ago.
Michael James: (30:51)
And the NBA is not unique in this. Other leagues are investing in the same way, both at the league level as well as across teams. They're all making the same choice, that this is an invaluable asset for us to have, we're gonna be smarter because of this, and we wanna invest more in it. I think that when you ask the question about culture, taking that as data-driven culture, I don't think you'll see too many places in sports now that differ on this point. I think everyone understands the incredible value that the resourcing, the technology in this space and investing in the space can yield when it comes to better decision-making.
Collaboration across the (sports league) industry
Satyen Sangani: (31:28)
And do you do a lot of cross-league work or do you collaborate with your peers in an equivalent function in other leagues?
Michael James: (31:35)
Even before the pandemic, we actually use the host get together at the different league offices amongst the folks that were dedicated to strategy and analytics to be able to just share, “Hey, what are the things that you're working on? What are the things that your teams are asking you for?” All of us are focused in different areas, and all of us have things that we do incredibly well, areas that we're looking to get into and would love to learn more, so that cross-league sharing is really important. It's something that’s privileged to have been in this particular type of function now for over a decade and really from the early stages of it. So getting to know a lot of people through the years. It's very easy to pick up the phone and call and say, “Hey, are you dealing with this particular thing? You're dealing with that thing?” I think, not unlike most of the world, the pandemic really threw a wrench into that, and I think we're all still trying to figure out how to get restarted some of the in-person things that we used to do...
Michael James: (32:31)
So, there's a lot less in-person interaction than there was pre-pandemic, but the ability to just chat, pick up the phone, spend 30 minutes with someone from across the different leagues, that hasn't changed. What I find in this space, particularly — although it's the only space I've been in — when it comes to the sales and marketing verticals of sports, is that everyone is very open to take a phone call…
Michael James: (32:55)
Share what they're working on. There's a collective desire to make this function work on the business side of sports, and a collective desire to make each other better, because there was a time, not that long ago. When the question of, “Do I need a CRM system, do I need a data warehouse? Do I really need a ticketing pricing analyst? What is this sponsorship analyst gonna do?” These were all questions that we faced and value that we had to prove. So when you're battle-hardened through those years, some of the folks that have been around the longest are the ones that are most willing to share because we all remember that time when it was not about actually influencing business decisions, it was merely an existential point of just proving our value...
Keeping the ball bouncing
Satyen Sangani: (33:41)
Yeah, and the ones who had to fight the battles are in some sense the most religious defenders of the guard, which makes total sense. Give us some examples of some of the problems you're working on right now, and give us some examples of what's hard in your job and what are the things that are more straightforward?
Michael James: (33:56)
What was hardest in my job: We set up this data strategy and analytics group two years ago, roughly, and we had very broad ambitions in terms of areas that we were going to staff up in for a variety of reasons, not the least of which is again in our transformation to becoming a direct-to-consumer company, the league office, we just needed more resourcing in the data space, so really, for about the first 18 months, my biggest problem was trying to get talent in a really hot marketplace. And I think that one of the things that's funny in sports is for so many years — again, in this space, I should say — for so many years, I was arguing for that next incremental resource and the value we can provide. Now you had the flip problem from mid-2021 to really the end of 2022, which was people understand the value and they wanna scale this and they wanna move fast and they wanna grow resourcing, and it's just really hard to acquire resources out in the marketplace. So, that was actually a big problem that I was working on through a lot of the initial phases of this new group; how do you target the right talent that sees the power that can come with working in sports in a competitive marketplace?
Michael James: (35:10)
Now, I think we've really locked our group and we've gotten to the point where we want to be pivoted a lot to some of the business problems that we face. Certainly, I think that we've spent a lot of time on what we call our fan data platform, but really our central fan data infrastructure that incorporates all of the data that we have access to, either directly through our partners or third parties, bringing that all into one place, we have had a central data warehouse at the league since about 2008. It's gone through a variety of different iterations, but the decision to move to the cloud in 2020 brought a bunch of challenges. Moving from what was legacy on-prem to the cloud, it takes a lot of effort, takes a lot of time building and not necessarily being able to immediately share results or outcomes, so that was a very big lift, and I always liken it to like when you build a house and it always seems fun: “Oh, I have this lot and I get to put a big house on it!” And then you start getting into the process and you realize, “Oh my goodness, there's so many decisions to make it down to like the light fixture in this room, and then all I gotta make sure that the contractor has all the answers that he or she needs and that...
Michael James: (36:30)
“I'm doing walkthroughs.” it becomes such an effort that you think about when I get to build a house, it's like, “Oh cool, I'm gonna get a new house.” It's gonna be great that it's taken up a lot of our time as well, because I wanna make sure we get that right, and that now that we have our final environment that's been live now for about 18 months, making sure that now we're getting value out of it. How can we socialize this with stakeholders, how can we make sure that we are effectively using our means of communication to share broad “what do we have,” and then also what can it be used for? And that's the most important piece: how is this powering business decisions today? How is this changing the way that we market? How is this changing our direct-to-consumer product or next-gen app, how is it impacting all of these different key strategic priorities that we have in the league. That's a lot of my day-to-day now, is really communicating out those wins and working with stakeholders to generate those wins.
Measuring performance to measure culture
Satyen Sangani: (37:08)
One of the ways in which people think about data culture is, interestingly enough, having people use data more often, but there's also the aspect of driving performance and people feeling treated fairly, and people feeling like they're objectively measured in terms of how they perform, and in some ways, I think that also is a way to drive data culture, but it can be really hard because in many cases, you're measuring people's own performance versus the organization's perception of their performance, and that's always a subjective conversation, but to your point, data can inform that decision in that process.
Satyen Sangani: (38:08)
And you're seeing it by the way, like not just in the sports field. Obviously the NBA has a collective bargaining agreement, but you also see it even in things like that, which are even more subjective. Like right now the Screen Actors Guild’s on strike or something like that. And we saw some of that in the New York Times. Tell us a little bit about the work that you've done to sort of help the league measure player performance and how that factors into, overall, the context of how these negotiations are held and handled and how you’re able to get to some of these economic answers around how to split the pie.
Michael James: (38:39)
I will say one of the benefits that we have as a league is that from the top we have a heavy focus on, in tech they're called OKRs, objective and key results. We call them our key metrics. But certainly through our corporate strategy group, which sits alongside us within our global strategy function at the NBA, they really heavily focus on our key metrics-setting throughout the organization. And with our NBA reports intranet site, actually our four key metrics as an organization are right at the top, including our pacing toward those metrics. And then each department at the NBA waterfalls off of those key metrics and sets their own key metrics, which are tracked either centrally or by department — but certainly with the data coming out of our central fan data asset. So there's that culture already within the league that would exist if we had a robust data strategy and analytics, it would be nice.
Michael James: (39:48)
Just part of our corporate strategy function is to set these types of metrics and to think about our performance in a very data-driven way. And certainly people, if you go out throughout the building, are gonna be conversant in what is one of those four company-wide key metrics that their group — maybe it's more than one — can impact. And then what are their own key metrics that they can impact? So we're really privileged to have that muscle already prebuilt. And then all of our focus is making sure that tree that builds up to it, the things that are leading indicators of the KM and whether or not we're going to hit it, that we're tracking those as well, and we're making sure we publicize those. Possibly something that is well-liked in the building or maybe not: We have the ability to subscribe people to dashboards and outputs.
Michael James: (40:28)
So for ones that we feel are particularly important, we'll go ahead and subscribe either our senior leadership team or particular department leads. We try to keep the flows to a minimum, but certainly we have a lot of different ways to make sure that data's getting in front of that, that data on our progress and how we're pushing toward those key metrics is getting in front of the key decision makers on an ongoing basis. It's definitely part of our culture. It's not something that we've had to work terribly hard on getting accepted. It is just something that, again, from the top at the NBA is something that we're very focused on, is that goal setting process, regardless of what the terminology for the goal is, the idea of having these data-driven metrics that we're pursuing.
Improving DEI in data
Satyen Sangani: (41:16)
Super interesting. As you think about sort of then furthering the field of data more broadly you're active in a group called the Women in Data and I believe you're the father of three girls. Is that right?
Michael James: (41:26)
That's correct.
Satyen Sangani: (41:33)
I think there are a number of reasons. Certainly the NBA has a very public diversity, equity, and inclusion push that we take very seriously. So there's an organizational reason, certainly through my three daughters. There's plenty of reasons to get involved. And I think also I've never really understood, certainly early on, there was this notion that like gender inequity in sports was like a manifest destiny problem. I never really got that. I didn't understand why that had to be. Certainly I've gotten to take a look at a lot of resumes off of a lot of job postings. There's no real reason why that has to be. Taking a look at the resumes that we receive, that doesn't necessarily have to be a foregone conclusion as an outcome. I've always been very supportive of any attempts to expand and broaden the pool of people that are interested in this industry.
Michael James: (42:26)
Even selfishly, it just makes total sense. It's very hard to hire people in a competitive marketplace. If we can get more people interested in sports business analytics, it just creates more of a pool to draw from. So it's an absolute no-brainer. And we've seen the results. I mean the data strategy and analytics team at the NBA is a majority female. Now I don't think that really should even need to be said as a surprise. I mean that's just hiring the best people. If you're really working hard on the pool of applicants that you have, that's an outcome that can entirely plausibly happen and we're very proud of it. But also at the same time, it's just the product of a lot of work and making sure that people that are interested in coming into sports strategy and analytics, we're trying to find anyone who has interest and bring them into the pool and make sure that everyone understands like there's no particular template for who gets to work in this industry.
Michael James: (43:22)
Anyone can work here. It's all about are you interested in working here and do you have the capabilities that we're looking for. Any efforts that we can take to broaden the pool to get more people interested in this, it's the right thing to do. It's also selfishly something that we're heavily focused on because we want more robust pipelines to be able to fill the roles in this space. If you look at the growth in sports business analytics across teams and leagues, you are only gonna be able to sustain that growth if you can continue to grow the pipeline. So that's a heavy focus of ours, whether it be through particular symposiums and conferences that are targeted towards women in sports, whether it's focusing on people of color, whatever it is, we're going to try to be broadening the pool that we can draw from. Because it's just a smart thing to do.
Satyen Sangani: (44:10)
For sure. And look, even though it shouldn't be a surprise, it's certainly not the norm. So obviously congratulations for both finding and recruiting and building such a consequential team.
Michael James: (44:21)
And I will say. It's going well on the home front. My 8-year-old is very, very into coding. Not necessarily coding related to data, but I take what I can get. So I've won with one of them.
Mike James’ top 5
Satyen Sangani: (44:33)
Most important question of this podcast is the great contribution of sports leagues to American discourses, who's the greatest player of all time. And so who are the top five players of all time in your view? And obviously this is not an NBA endorsement, this is just a Mike James [list]. Like tell us who the top five are.
Michael James: (44:55)
Well, since I'm not qualified to give an answer in this space, I'm gonna give a very Mike James answer in this space. My favorite player of all time is of course Jeremy Lin. And I think getting to watch those games in 2012 as a Harvard alum was one of the thrills especially because it was very early in my time at the NBA, just the serendipitous timing. I might say that that era, Jeremy Lin was one of the five greatest players of all time [laughter] I think people would disagree on anything outside that era, but certainly that's been one of the top five thrills as an NBA fan was to watch that. I tend to agree with the consensus without taking a stance on one versus two or one versus two versus three with MJ, Kobe, and LeBron. So I'll mention them. And then for my fifth, it's hard for me to pick anyone other than Shaq because I grew up in Jacksonville, so my nearest NBA team growing up was the Magic. And certainly they took off when Shaq joined, and being there during the 90s when that team really took off with him being there, I think that was pretty incredible. So I'm giving a very subjective, biased version of a top five.
Satyen Sangani: (46:05)
Is there any other version of a top five?
Michael James: (46:08)
If you got folks that were heavily on the basketball side, they could give you a very cogent and intelligent take on different stats or achievements or things that they feel separate out the top five. But I'm more focused on the players that I enjoy watching and certainly you enjoy greatness, but also you enjoy those parochial folks that are of interest to you as well.
Satyen Sangani: (46:31)
For sure. It's very subjective and there's a lot of memories associated for people that are fans. Mike, thank you for taking the time. This was really a fun conversation and I know — nott just because of the topic, which I think obviously alot of people are interested in, but also because of how you do your work — I think people would've been super informed and would've picked up a few tips. So, thank you for that.
Michael James: (46:49)
Great to be here. Thank you for having me.
Satyen Sangani: (46:57)
As I mentioned, Michael's tactics remind me of those of another data radical like General Stanley McChrystal. Michael knows the value of regular communication and transparency. The NBA's TMBO meets often to share best practices and enforce them so all teams can capitalize on one team's data victory. It's no coincidence that Michael spent a decade on TMBO before rising to chief data officer. He's an expert collaborator, so he knows that the best results come from business leaders with a talent for hypotheses. Those who can use intuition to frame the right question in data terms, have a serious edge, and it's clear that every organization could benefit from a TMBO model. While some healthy competition is great, we can all get better by learning from the All Stars. Thanks for listening, and thanks, Mike, for joining today. I'm Satyen Sangani, CEO of Alation. Data Radicals, keep learning and sharing. Until next time.
[music]
Producer: (47:51)
This podcast is brought to you by Alation. Does data governance get a bad rap at your business? Today, Alation customers wield governance to drive business value, increase efficiencies and reduce risk. Learn how to reposition data governance as a business enabler, not a roadblock. Download the white paper Data Governance Is Valuable. Moving to an Offensive Strategy.
Subscribe to the Data Radicals
Get the latest episodes delivered right to your inbox.