What’s the Difference: Quantitative vs Qualitative Data
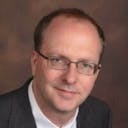
By Jim Barker
Published on October 12, 2022
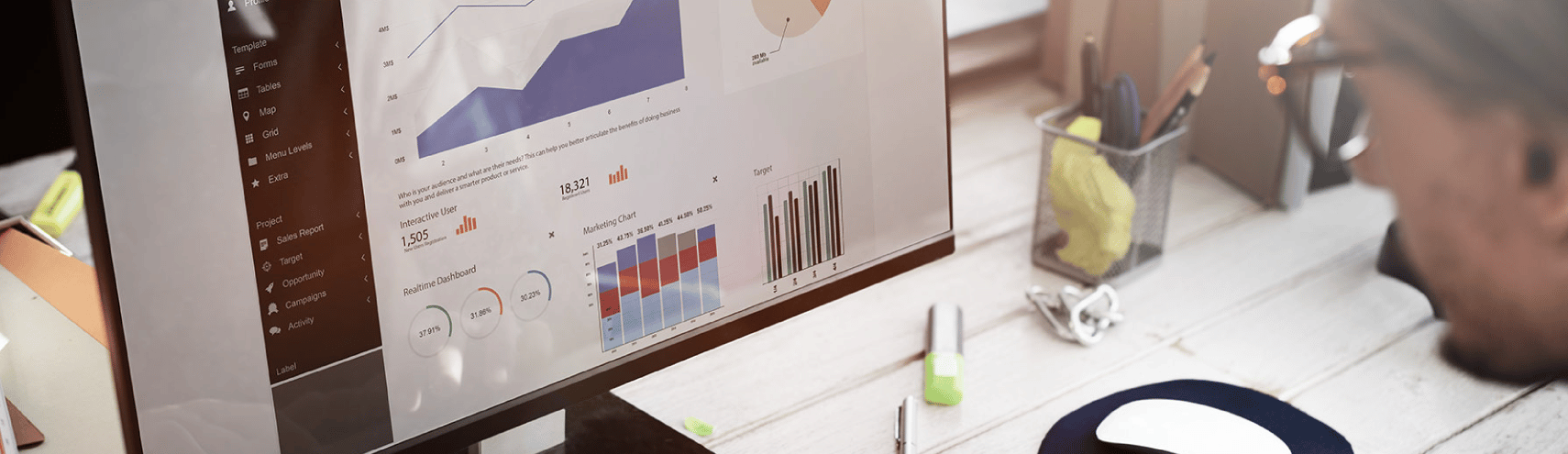
Companies collect and analyze vast amounts of data to make informed business decisions. From product development to customer satisfaction, nearly every aspect of a business uses data and analytics to measure success and define strategies. A big part of data analysis includes understanding what’s essential about collected information.
While quantitative and qualitative data are often used interchangeably, they have significant differences that impact how you collect, analyze, and use them. When choosing between qualitative and quantitative data, think about what you want to learn. You might choose to use quantitative analysis to determine what happened. However, if you want to understand why something happened, qualitative data can deliver a more robust picture.
So what are the key differences, and what should data users know about them? This blog post will break down how to use quantitative and qualitative data, as well as some common methods for analyzing the data to uncover quality insights.
What Is Quantitative Data?
Quantitative data is data that expresses quantity, amount, or range. Data users will often set boundary limits to this sort of data, and may apply arithmetic operations to it and aggregate it for analysis. Quantitative data may be understood as variables in an equation, and these variables can be independent, dependent, or even extraneous.
Some examples of quantitative data include:
Counts or units, stored as raw numbers
Currency amounts, which are frequently stored as decimal
Percentages
Ratios
Measures of central tendency
It is important to take into account that not all numeric data can be part of a calculation or aggregated. These terms that cannot aggregate, like a percentage, are often called non-aggregatable metrics.
What Is Quantitative Data Analysis?
Quantitative analysis can take two forms: the traditional business analysis of numerical data, or the more academic quantitative analysis.
Traditional business analysis uses numerical methods to paint a picture, often through numerical methods, like statistics. Typically, people use one of the following two types of statistical analyses:
Traditional Business Quantitative Analysis is the process of collecting and evaluating measurable and verifiable data such as revenue, market share, and wages in order to understand the behavior and performance of the business (CFI).
One of the most powerful quantitative tools is segmentation. Segmentation is the technique of collecting data across two axes, such as details about the customer, and the specific goods or services purchase. Analysts then review the relationship to place the customer in different segments to better understand your customer base.
A good example of segmentation from the early days of analytics is Postal/Zip Code Analysis. In the 1980s, retailers would collect data at the Point of Sale, otherwise known as a cash register. Generally they would only capture a zip code due to technology limitations. This data could then be aggregated and analyzed to answer the following questions for any given retail location:
Where do our customers live?
What products do people buy who live in close proximity? What products do people buy who live farther away?
How often do local customers purchase? How does that compare to non-locals?
Given the above, where might we need more stores?
Another example of segmentation is market-basket analysis, which explores the types of products consumers most often purchase together. Data leaders at retailers can use these insights in many ways. When managers of physical stores understand market-basket patterns, they can place products on shelves more thoughtfully to drive sales. Communication managers can retarget loyalty members with more attractive coupons. And so on.
Academic Quantitative Analysis represents the next chapter in zip code analysis; this form of analysis focuses on the interplay between variables after they have been operationalized, allowing the analyst to study and measure outcomes (Quantitative and statistical research methods: from hypothesis to results, Bridgmon & Martin, 2006.). This method differs from traditional business analysis in that it normally will include hypothesis testing around a single research question; by contrast,the traditional business approach focuses on reviewing outcomes and prescribing actions or new questions.
What Is the Role of Statistics in Quantitative Data Analysis?
Statistics is at the heart of quantitative analysis. It generally falls into one of two categories: Descriptive statistics or inferential statistics.
Descriptive statistics summarizes a set of data, either an entire population or a random sample. This table is an example of descriptive statistics, ith mean and standard deviation included:
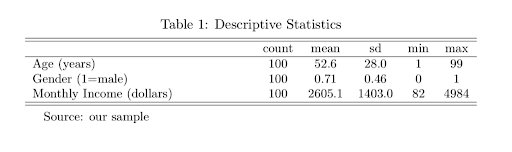
The goal of descriptive statistics is to tell a story that summarizes data in aggregate.
While descriptive statistics captures what is, inferential statistics predicts what could be, and typically has one of two purposes:
Make estimates about populations as dependent variables change, or
Test hypotheses in order to draw conclusions about populations (for example, the relationship between Lifetime Value and Annual Revenue).
Two of the most common types of inferential statistics are:
Regression analysis. This is the act of evaluating across a population how one variable will change with respect to another. Linear regression is most common and is based on changes to an independent variable based on the values of its dependent variable.
Hypothesis testing is one type of inferential statistics that is used to ask a question and test the answers. (For a refresher on hypothesis testing, this cuemath summary is very helpful.)
This table from cuemath summarizes these statistics approaches nicely:
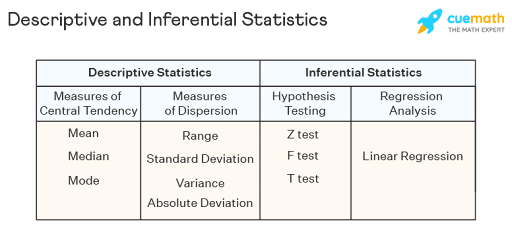
Advantages of Quantitative Data
Quantitative data comes with several advantages, including:
Closed-ended questions: Analysts can gain insights by using a few discrete variables, like asking yes/no questions.
Easy to analyze: Data users can easily use mathematical models to analyze quantitative data.
No interpretation necessary: Numbers are objective, so there is little concern about misunderstanding them.
Not always expensive: Free or low-cost survey tools can make gathering information cheaper.
Easy to automate: Technologies, such as tracking software or social media analytics, offer consumable information without users having to engage in manual tasks.
Disadvantages of Quantitative Data
Although quantitative data is valuable, it also has several limitations or disadvantages, including:
The data you need for the analysis needs to be:
Available
Of high quality
Trusted
The tools to do the analysis quickly and efficiently need to be understood
The analyst needs to have a good degree of business acumen to provide the analysis
What Are Quantitative Data Collection Methods?
When collecting quantitative data, it’s important to collect as many data points as possible to ensure that your mathematical analysis gives you a meaningful answer.
Some common methods to obtain quantitative data include:
Systems Data: As businesses operate, they collect a wide range of data spanning sales data, financial data, and logistics & transportation data, all of which can be used for a wide range of operational and analytical purposes
Surveys: Use a questionnaire to gather information. For example, you might send out a customer survey asking people to rate their satisfaction with your product or service.
Analytics: Analyze data to understand how people interact with products and/or services. For example, you might track how people interact with your company’s website.
Experiments: Use two data sets based on control and test groups, then compare numbers. For example, you might use A/B testing to compare customer reactions and conversions to decide what website copy works best.
Manipulation of Preexisting Data: Change what you have without actually creating new data; for example, you might change the numbers in a spreadsheet to see what could happen if people took a different action.
What Are Common Quantitative Data Analysis Methods?
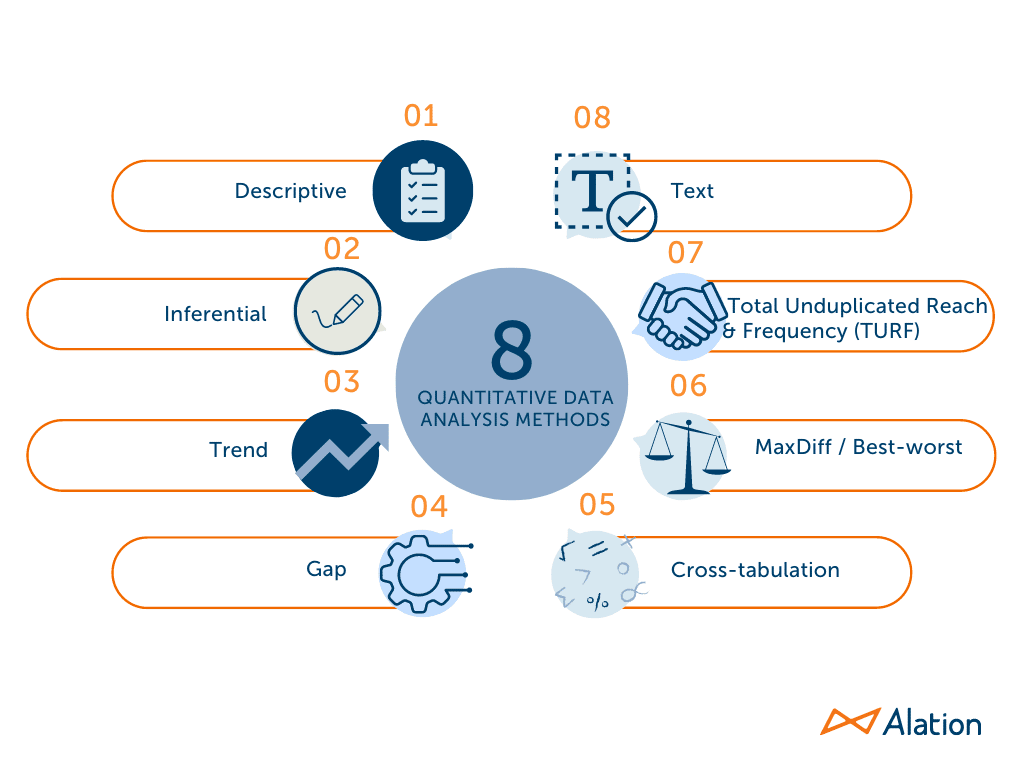
By understanding the different quantitative analysis methodologies, you can choose the right one for your business’s needs.
Descriptive
A descriptive analysis is a summarization of data points that describe the data. Statisticians like to describe these in terms of central tendency or spread, while non-statisticians look for narratives to describe what the data means. A simple example of a narrative this could be a descriptive column on a report that reviews sales 12 months ago and last month and states: Sales has grown from $1.2M in FM2021-12 to $1.4M in FM2022-12 based largely on growth in appliances.
Inferential
Inferential analysis is the ability to draw conclusions based on reviewing samples of data What’s an example of inferential analyis? Here’s a a common one: when interest rates rise, house sales decrease. Analysts infer a connection between the two variables: “Because the cost of a mortgage is higher, fewer people are buying homes.”
Analysts use inferential analysis to to explain why something happened. For example, data users may compare sales between two different industry verticals to create an ideal customer profile.
Trend
Trend analyses review data collected over time to help predict the future. For example, an age demographic’s purchasing decisions can be tracked to see if that group will likely purchase a product in the future.
Gap
In gap analysis, past and current state data is compared to evaluate performance or make decisions about what needs to be done to fix a problem. For example, past weekly sales employee hours can be compared to current weekly sales employee hours to determine whether more employees are needed.
Cross-tabulation
A cross-tabulation or contingency table groups multiple variables together so that mathematical correlations are easier to find. For example, data about a person’s age and the time of year when they purchase the most items can be aggregated to gain visibility into how people shop for the holidays.
MaxDiff / Best-worst
With a MaxDiff, you analyze how people responded to a survey’s “most important to least important” scale question by creating a mean score for each point on the scale to determine the order of preference. This can also be used when doing market research to understand how important a new feature would be to customers.
Total Unduplicated Reach and Frequency (TURF)
The TURF analysis methodology helps assess a combination of products and services by reviewing the number of customers reached in conjunction with how often the communication source reaches them. It’s usually used in market research and often combined with a MaxDiff analysis. For example, when a business is trying to decide which of three services to prioritize for customers, statistical analysis can be used to identify the most popular one.
Text
A text analysis uses statistics and automation to draw inferences by looking at the number of responses containing a word or phrase, the respondents’ grammar, or themes within responses. For example, a text analysis can be used to identify key emotional themes across customer satisfaction surveys.
In summary, quantitative data offers objective insights. But it often fails to answer some important questions, mainly, why? Qualitative data is key to understanding why things happen. To learn more, read on.
What is Qualitative Data?
Qualitative data provides labels or numerical values for a characteristic of an element (Statistics For Business and Economics. Freeman, Anderson, Sweeney, Williams, Shoesmith. 2006). More generally, it is information collected about human behavior that describes things in terms of words rather than numbers. It uses people’s feelings and experiences to understand the underlying reasons for why an event occurred.
This is not restricted to surveys or questionnaires; qualitative data can be analyzed whenever data is collected in free-form text. Some examples of this type of data include:
Call logs from customer service software
Notations from social media posts
Product descriptions
Ordering instructions
Open-ended questions from interview & surveys
What is Qualitative Data Analysis?
Qualitative data analysis involves a researcher reviewing information from interviews and questionnaires and applying labels and numerical values based on repeated words, phrases, or sentiments. Their goal is to find and connect patterns and themes around various qualities or characteristics.
An example of a company that has built its reputation on this type of data is Gong. Gong collects the details in call logs, meeting transcripts, and other free texts and places numerical values on this to show the progression in sales cycles to drive the sales pipeline. Their marketing message is reflects this: “Stop losing winnable deals by turning qualitative data into actionable intelligence.”
What Are The Advantages and Disadvantages of Qualitative Data?
Qualitative data offers subjective insights about an underlying cause, but it can be time-consuming to analyze.
Advantages of Qualitative Data
Qualitative data comes with several advantages, including:
Open-ended questions: Without defined variables, you can answer the how and why behind events.
Fast to collect: It’s easy to run a survey and get an abundance of answers within a short timeframe.
Details: Data users can get more information about people’s feelings and opinions in their own words.
Deeper insights: People’s opinions and attitudes help to explain their behaviors.
Disadvantages of Qualitative Data
Qualitative data comes with some disadvantages, as it can be:
Hard to collect: If analysts require interviews to get information, finding the right respondents can be difficult.
Time-consuming: One-on-one interviews take time, and analyzing results isn’t easily automated.
Resource intensive: Leaders need to have people who know how to review the information to extract meaningful insights.
Difficult to quantify: Human emotions and opinions don’t always translate to objective numbers.
Prone to bias: People create the questions and interpret the responses, so their biases can impact the process.
What Are The Qualitative Data Collection Methods?
Qualitative research includes both structured and unstructured approaches.
Some examples of structured approaches include:
Focus groups: A group of participants discuss a topic together and answer questions about it. For example, a focus group lead may ask the group how they would feel about a new product when conducting market research.
Case studies: Talk to a customer about how they use a product or service to understand their perception of its value. For example, businesses may ask a valuable customer how they use a product and what they would like to see in the future; these answers then inform how they update the product.
Some examples of unstructured approaches include:
Observation: This entails watching how people interact with their environment. Market researchers may observe people shopping in a store to see which displays attract their attention.
Interviews: This is a one-on-one conversation where questions are asked to understand the person’s motivations. For example, when deciding on a company’s branding, marketing leaders may ask someone in a certain age demographic what types of branding appeal to them.
Document analysis: This entails reading through different documents about a topic and looking for common themes. For example, business leaders may read multiple industry reports from various analysts to inform how they develop the product and market its value.
What are the Types of Qualitative Data Analysis Methods?
Qualitative data analysis is often more challenging and time-consuming due to inherent complexity; it’s important analysts take time to understand the the data to deliver accurate and helpful conclusions.
Content
Content analysis is a subjective interpretation of data that includes the following steps:
Preparing data
Defining the unit of analysis
Creating categories
Establishing a coding scheme
Testing the coding scheme
Coding the text
Reviewing for consistency
Drawing conclusions
Reporting findings
For example, content analysis can be used to find correlations and patterns across focus group answers to make decisions about product development.
Discourse
Less systematic than content analysis, discourse analysis enables interpretation by exploring the meanings that language produces. This includes the details within the text and contextual knowledge about how people use language. For example, analysts use discourse analysis to understand how people communicate in interviews; this helps them obtain insights on how to write compelling marketing materials for a particular audience.
Grounded Theory
Grounded theory analysis uses transcripts of interviews to look for repeated themes by coding them with keywords and phrases to create a concept hierarchy. Based on respondents’ explanations or interpretations, companies use this method to understand what people’s social actions, interactions, and experiences mean. For example, grounded theory analysis can be used to correlate two different populations, like age and geographic demographics, to understand a new market.
Narrative
Narrative analysis revolves around the premise that stories are functional and purposeful. People use stories to organize their ideas and understand their lives. The four narrative analysis frameworks are:
Structural
Functional
Thematic
Dialogic/performance
For example, narrative analysis can be used to understand how someone’s relationship to an organization’s branding impacts their willingness to buy from them.
Thematic
A thematic analysis examines themes or patterns in data. It requires less theoretical and technical knowledge, and as thus more accessible. The three thematic analysis types are:
Coding reliability
Codebook
Reflexive
For example, you can use thematic analysis of social media users to understand how a customer segment feels about a competitor.
Interpretative Phenomenological Analysis (IPA)
An IPA explores people’s responses in relation to their lived experience; it seeks insights into how someone would understand an event based on a given context. While grounded analysis tries to understand how people process events, IPA takes a philosophical approach focused on the essence of an experience. For example, IPA can be used for insights into how customers at a specific restaurant location felt about the service provided.
Natural Lanugage Processing
Natural language processing (NLP) is a machine learning analysis technique. It leverages algorithms to analyze textual data. Common examples of NLP include email filters, spell-checkers, and translation engines. When Google auto-completes a phrase or your phone pulls a voicemail transcript, NLP is responsible.
NLP is evolving fast. Many researchers are excited by its potential for supporting qualitative research. When NLP methods are incorporated into such analyses, scientists can cut costs, increase sample size, and shrink timelines.
What are the differences between quantitative and qualitative data?
With quantitative data, numbers offer objective metrics. Qualitative data, by contrast, provides information about people’s feelings and opinions, which is more subjective. Whereas quantitative data communicates an event’s frequency, qualitative data can uncover why it wasn’t more or less frequent.
For example, a business may consider discontinuing a product. How can the analysts use data to inform that decision? Quantitative data helps them understand how many people purchased the product and the revenue earned, whereas qualitative data would reveal why people didn’t buy it. While quantitative data reflects a new event (people buy less) quailtiative data uncovers why it happened. Through surveys, the business may learn that the product was missing an important feature and decide to update it instead.
Quantitative data can also help determine whether changes need to be made and whether or not those changes would be effective or ineffective.
How Alation helps businesses organize their qualitative and quantitative data
With Alation’s Data Catalog, companies can unlock the power of both qualitative and quantitative data. Our Data Catalog supports data analysts by ingesting metadata and utilizes machine learning alongside NLP to create a powerful, searchable hub.
With Alation, leaders can achieve data-driven business outcomes quickly, as they can quickly discover the relevant qualitative and quantitative data within the appropriate business context.
Curious to learn more?
- What Is Quantitative Data?
- What Are Quantitative Data Collection Methods?
- What Are Common Quantitative Data Analysis Methods?
- What is Qualitative Data?
- What Are The Advantages and Disadvantages of Qualitative Data?
- What Are The Qualitative Data Collection Methods?
- What are the Types of Qualitative Data Analysis Methods?
- What are the differences between quantitative and qualitative data?
- How Alation helps businesses organize their qualitative and quantitative data
Contents
FAQs
With quantitative data, numbers offer objective metrics. Qualitative data, by contrast, provides information about people’s feelings and opinions, which is more subjective. Whereas quantitative data communicates an event's frequency, qualitative data can uncover why it wasn’t more or less frequent.