Why Your Data Governance Strategy is Failing
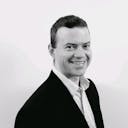
By Aaron Bradshaw
Published on October 5, 2021
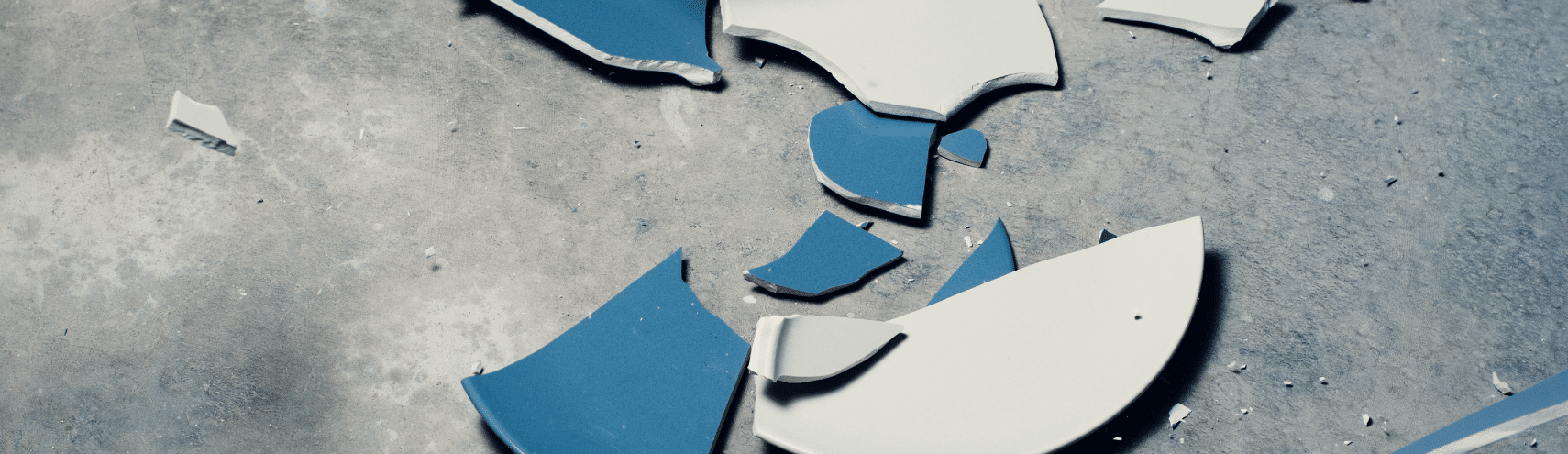
What is data governance and how do you measure success?
Data governance is a system for answering core questions about data. It begins with establishing key parameters: What is data, who can use it, how can they use it, and why? Answers will differ widely depending upon a business’ industry and strategy for growth.
But what comes after these parameters are set? What does success look like? And how can leaders ensure their governance initiatives contribute to larger organizational goals?
The first step towards a successful data governance strategy is setting appropriate goals and milestones. These goals must also be SMART— Specific, Measurable, Attainable, Relevant, and Time-based. This best practice has been the foundation of the world’s most profitable organizations across all industries and business sectors for decades. Yet, so many companies today are still failing miserably in implementing data strategy and governance protocols.
Why is your data governance strategy failing?
To measure failure vs. success, governance leaders must actively monitor all governance efforts. According to the Gartner report, The State of Data and Analytics Governance Is Worse Than You Think, approximately 80% of businesses readily acknowledge that high-quality data governance is essential to achieving long-term business goals, objectives, and outcomes.
However, about 50% of those surveyed also admit that they do not assess, monitor, or measure their data governance systems. Perhaps even more alarming: fewer than 33% expect to exceed their returns on investment for data analytics within the next two years.
Gartner further estimates that 60 to 85% of organizations fail in their big data analytics strategies annually (1). If this survey is trustworthy, then most business leaders are already fully aware of the critical importance of a detailed and well-thought-out data governance methodology.
So, why is YOUR data governance strategy failing? The answer may be more straightforward than you think.
Common data governance challenges
The main reasons that a company’s data strategy and governance protocols fail to deliver are somewhat universal, regardless of the industry sector. For organizations to succeed in monitoring, managing, and optimizing their data resources, they must always keep the importance of their data-driven initiatives at the top of all strategic planning agendas.
Without a doubt, no company can achieve lasting profitability and sustainable growth with a poorly constructed data governance methodology. Today, all companies must pursue data analytics, Machine Learning & Artificial Intelligence (ML & AI) as an integral part of any standard business plan. The inevitable trend towards the digital economy leaves no room for companies that do not adhere to this core principle. Yet there is often lack of awareness of the trustworthiness (or lack thereof) of the data that these algorithms are being trained on.
It is commonplace for a company to create an enterprise data governance strategy that fails to even consider the end user. Most data governance initiatives focus on outcomes and compliance — rather than efficiency, scalability, or ease of use for the average human. These divergences of focus can lead to consumers feeling bogged down by overly complicated processes or leadership teams being unable to see initiative investments reap the desired rewards of their predictive business success metrics. (1).
Over time, this failure in strategy will lead end users to sidestep these practices completely. They may simply move and copy data without going through all of the necessary protocols related to data security and other best-practice standards. Below is a list of primary reasons covering why implementing good data governance policies can be so tricky.
Top 3 Roadblocks to Successful Data Governance
Roadblock #1: Governance Committee Challenges
Lack of focus on the right areas. In many cases, data strategy and governance protocols fail because the governance committee members focus more on their day-to-day tasks rather than the defining and monitoring of company-specific metrics, rules, and KPIs.
Lack of commitment. Governance strategies can fail when the company is more focused on talking up the ideals and objectives of the project without fully committing to its success. Management essentially talks the talk without walking the walk.
Improper priorities. When the committee faces one of two rather extreme scenarios, governance strategies fail. Either the program is so complex that substantive issues remain unaddressed, or the system becomes buried in trivial details that lack significance or relevance. One typical example of the latter is the use of “data cops” to police their lower-level end users.
Too much on their plates. It is also common for data governance strategies to fail because committee members are responsible for far too many projects. The committee often spends more time arguing than making progress towards creating favorable outcomes and results.
Incomplete data. If managers rely on incomplete or unproductive metrics, this can obstruct strategy. For example, it may be helpful to track specific daily activities or benchmarks for all data-related processes.
Too much time discussing the menial before getting to the meaningful. Numerous committees spend hours deliberating over every word in a Glossary definition, then 6 months down the line leaders complain there hasn’t been enough value shown
Roadblock #2: Data problems and inconsistencies
Inconsistencies in expectations can create enormous negative issues regarding data quality and governance. With so many missed signals and crossed wires, employees begin throwing their hands up in the air out of frustration.
A lack of a shared vocabulary across different departments within the organization can also lead to inconsistencies in data reporting, making it more difficult to launch or adhere to an effective data governance strategy. The negative feedback loop that follows creates even more confusion and generates even more insufficient insights.
A typical example might involve different employees using differing calculations for essential business metrics. The situation worsens when each employee performs these calculations in standalone none-systematic solutions that are not synced or disconnected from the company’s centralized information hub.
Roadblock #3: Silos Breed Misunderstanding
A data silo is an island of information that does not connect with other islands. Typically, these data silos will prevent two-way flows of data outside and inside of the organization. This disconnect often precludes the company from performing analytical reporting tasks efficiently and accurately.
Silos of understanding represent the same concern, but symbolize how people’s understanding and trust in the data suffer if they work in isolation. If the same piece of information means 5 things to 5 people, no BI report, Analysis or model will have the same meaning!
A big reason a data governance strategy fails is that it lacks a solid or robust knowledge hub to house its information and accumulated metadata. This disconnect between departments can lead to many different issues related to ineffective communication, misinformation, and poor decision-making.
Data governance and AI
Absence of data governance is likely to lead to poor quality data. Bad data can spell trouble for AI systems. One common issue arises from the misinterpretation of raw data derived from different data sources — including those siloed in dispersed physical archives, cloud-based servers, and other digital databases.
This situation is also common when the company lacks a unified way to store its information, or if the company lacks data quality checks on data used to run AI and ML. The lower the quality of the data going into a model, the less reliable the output is going to be – and this can open up risk of regulatory fines for biased models.
The result of this scenario is that employees with different skills and tools will read the data differently, which leads to incorrect inferences and conclusions. These inconsistencies can then lead to poor decisions or even create security issues related to incorrect data usage in various reports and programs.
Aligning data strategy and governance with business outcomes
The strategic goal of data governance is to align decisions-in-the-making with targeted business outcomes. This connection should be present at all levels of an organization, from a department and division level to the top-tier leadership. The entire organizational hierarchy must thoroughly understand how data initiatives tie to the company’s overall profitability and success. To achieve this objective, forward-thinking enterprises align data strategy with governance.
How to Align Data Strategy with Data Governance: Key Steps
Develop measurable KPIs.
Connect the KPIs to the relevant data resources to achieve the desired targets.
Ensure that both the metrics and the assets are linked clearly to stakeholder and company value.
Establish a data governance program that drives business value by aligning team roles to KPIs.
The data quality management team in coordination with the data governance committee will then familiarize the organizational hierarchy on how the enterprise data governance strategy will help each department achieve their related goals and objectives.
During these conversations, another important goal of the data quality management team is to nurture employee buy-in and recruit peer support. In doing so, the chances of long-term success rise exponentially.
The failure to govern data is hurting organizations of all sizes, industries, and business sectors. The irony is that most executives are acutely aware of the problem—but they aren’t doing much about it. Having a detailed plan of action from the very beginning streamlines the entire process.
Picking the Right Data Governance Tools
Every enterprise will eventually face challenges regarding its data governance policies. Issues involving data quality, visibility, and security can be both highly complex and somewhat expected. Furthermore, many organizations in certain industries face a growing onslaught of regulatory scrutiny from organizations like HIPAA, SOX, GDPR, and CCPA. Is it any surprise that decision-makers are feeling overwhelmed?
At Alation, we help business leaders develop an effective data governance operating model and help them to better understand how data governance decisions should be made. We empower them to amplify the importance of an optimal data governance strategy throughout the organization while also providing guidance on how best to maintain this newfound enthusiasm for years to come.
With standardized, documented processes like the RACI matrix, data quality leaders will facilitate interdepartmental collaboration, communicate confidently about their data use protocols, and proactively resolve potential conflicts more quickly.
Alation’s active data governance framework delivers expertly governed data in real time by focusing on intelligent data policies, access controls, and privacy-enhancing technologies (PETs). By partnering with Alation, organizations will reap the following rewards:
Data discovery and classification: Alation’s automated data discovery platform saves time and effort by classifying and tagging global policies. These capabilities enable quick access to the information you need without going through a tedious manual inspection process — with faster turnaround times than ever before. Automated sensitive data discovery helps businesses find hidden assets of valuable insights quickly while also reducing their risk exposure.
Consistent data privacy: Alation has made it much easier for data teams to control the handling and employee access of sensitive data in a Snowflake data ecosystem. With one policy, they can apply the same settings across individual cloud instances without starting from scratch every time. This framework includes dynamic masking techniques, such as:
K-anonymization
Format-preserving masking
Joins on masked data
Rounding
Differential privacy
Randomized response
And conditional logic capabilities that make implementing these controls easy while still protecting the most sensitive information.
Data monitoring and auditing: Data is a powerful tool for any company, but it can also be dangerous if sensitive information falls into the wrong hands. Alation’s automated data audit trails provide peace of mind for companies managing an overwhelming amount of valuable data. Our system helps track what data was accessed, by whom, when, and for what purpose. Meanwhile, data fingerprint capabilities can identify changes made over time in case anything questionable pops up down the line.
The reasons for data governance strategy failures are more commonplace than many business leaders might imagine. As the premium provider of professional data quality management and data governance consultancy services, Alation has the expertise and years of experience to help organizations of all industries and business sectors achieve success. For more information on developing an optimized data governance strategy, contact Alation today.
Gartner, “The State of Data and Analytics Governance Is Worse Than You Think” Saul Judah, Andrew White, 19 June 2020GARTNER is a registered trademark and service mark of Gartner, Inc. and/or its affiliates in the U.S. and internationally and is used herein with permission
- What is data governance and how do you measure success?
- Why is your data governance strategy failing?
- Common data governance challenges
- Data governance and AI
- Aligning data strategy and governance with business outcomes
- Picking the Right Data Governance Tools