Data Mesh: Evaluating Your Organization's Readiness for a Decentralized Data Future
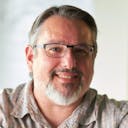
By Jay Piscioneri
Published on July 13, 2023
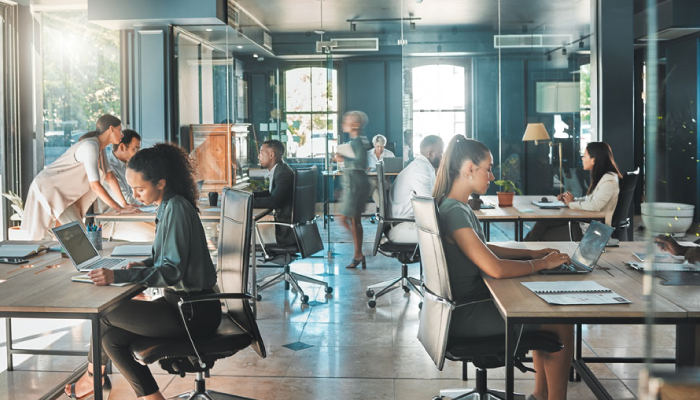
Article reposted with permission from Eckerson
ABSTRACT: Data mesh is a new paradigm for fulfilling the promised value of data. But it’s not for everyone. Learn how to assess if you’re ready for data mesh.
Sponsored by Alation
Read the first installment in the blog series: Data Mesh: The Sky is Not Falling
Data mesh offers a new paradigm for fulfilling the promised value of data. It decentralizes both data ownership and the data itself, shifting them toward the functional domains that create and use data to run their business. But data mesh is not for everyone. An organization must have lots of highly diverse and distributed data. And companies must work within the bounds of what their culture, technologies, and resources will allow. In this article we’ll review the main principles of data mesh and propose criteria by which to evaluate your organization’s readiness for the changes it prescribes.
The Four Pillars of Data Mesh
The four pillars of data mesh, domain ownership, data as a product, the self-serve data platform, and federated computational governance, are well documented in books and articles by Zhamak Dehghani. So, we’ll briefly review them below.
The Pillars of Data Mesh

Domain ownership. Data mesh transfers ownership of data from centralized data teams to distributed domain-oriented teams. A domain team is close to key business processes, knows the data the domain produces, and the analytics that its stakeholders need to solve problems and capitalize on opportunities. In data mesh, they are responsible for storing, cleaning, cataloging, and making their data available to its consumers in the forms they need.
Data as a product. Most organizations bring product thinking to their application development efforts. They have well-established ways of learning what stakeholders need, validating designs, and developing functionality that they improve iteratively. Data mesh applies this product mindset to data in the form of a data product. Data products consist of more than just data. They include code to collect and transform data and enable managed access through APIs. They include metadata that describes the product such as schema, semantics, and quality metrics.
Self-serve data platform. Domain teams need a self-serve platform to deliver and manage data products. They need to provision storage and compute infrastructure, build, deploy, and manage data product versions, clean and transform data, provide secure data access, and comply with policies and regulations. And they need to do all these things autonomously to prevent new bottlenecks from forming. Data consumers need the self-serve platform to find and access data products. They need to understand a data product’s purpose, the timeliness of the data, and its trustworthiness. These attributes are part of the data product itself, but the platform needs to surface them for consumers to evaluate how well a product meets their needs.
Federated computational governance. Data mesh calls for a federated body consisting of representatives from domain teams and members with global data responsibilities such as regulatory compliance and managing quality. They work together on common concerns such as what constitutes quality, data classifications, modeling data that span domains, and standards for data product metadata. While these are familiar data governance concerns, with data mesh, it’s the domain teams that enforce governance standards and policies by building computational, i.e., automated controls and observability into their data products.
Who is Data Mesh For?
Data mesh is not for every organization. You need sufficient data size and complexity to justify the investment in a data mesh program. Organizations with more of the following characteristics will find the data mesh journey less fraught with peril.
Big enough and complex enough. Data mesh is overkill for smaller organizations. To justify the effort and cost of transitioning to data mesh, an organization’s data must have sufficient mass and complexity. For example, a national restaurant chain with hundreds of locations, multiple customer constituencies, marketing strategies, suppliers, talent pools, and operating schedules is big and complex enough to potentially realize benefits from data mesh. An individual owner-operated restaurant is not.
Time and money. It takes time to make the fundamental changes that data mesh calls for. It also takes money to shore up the technical talent and tools needed to build data products, a self-serve data platform, and the global guardrails of federated governance.
Decentralized decision-making. Organizations that tend to empower employees with decision-making authority within a framework of accountability will experience less friction in implementing data mesh. By contrast, organizations that make decisions through a multilevel approval process may find data mesh principles harder to embrace because they run counter to their existing culture.
Active executive support. Data mesh, like any change initiative, requires active executive support to be successful. This means clear, consistent messaging about what the end state looks like, the challenges to overcome, and the opportunities to seize. It also means material support through allocation of resources and a commitment to the long haul.
Strong data culture. Many organizations are committed to making decisions and driving actions with data but are hampered by legacy technology and processes. These organizations are better prepared to transition to data mesh. They understand what they can achieve if they remove the barriers that limit their ability to derive insights from data. They also recognize how effective data governance is critical for trustworthy data and have mature processes in place to define and manage data quality.
Collaborative. Developing data products is a collaborative process between domain teams and data consumers. Organizations whose cultures espouse and reward cross-functional collaboration are therefore in a better position to adopt data mesh than those that struggle with collaboration and operate in silos.
Ample technical skills. Designing and building data products is a technical endeavor requiring software engineers, data engineers, infrastructure engineers, and data governance experts. Companies with a large technical staff have more to work with in assembling domain data teams. This gives them flexibility to recruit team members with the right skills and a spirit of bold curiosity for the adventure ahead.
Agile practitioner. Many of the principles and practices of data mesh align with the agile development methodology. Data mesh and agile both rely on empowered product teams, collaboration, iterative development, and strong product owners. So organizations that practice agile development already have experience with the skills and methods they’ll need to deliver a data mesh.
Not all of these criteria are of equal importance. The table below shows three factors that should be weighted more heavily than the others.
Data Mesh Fit Criteria and Importance

Most important is the size and complexity of your data. If you’re dealing with the volume, velocity, and variability of big data, then consider data mesh. However, you should not pursue data mesh if you only have a handful of sources, one or two data formats, and low transaction volumes.
If you’ve got the data challenges that data mesh targets, then time and money is your next concern. Doing data mesh requires material support and a commitment to the long haul. Start small with a limited pilot that shows the value data mesh will deliver. If that wins approval for further support, expand the program incrementally.
While time and money are very important, so is your organization’s culture regarding decision-making. To be nimble and responsive, domain teams must be empowered to chart their own course, change priorities, and adopt new development methods. If that goes against the grain of your organization’s culture, consider how you can overcome resistance or whether the cultural immune system will see a foreign body that it must eliminate.
Conclusion
Data mesh’s transformative potential lies in decentralizing data ownership and management. Therefore, you should evaluate your organization’s readiness for the people, process, and technology changes it demands. Having lots of data from many sources in many formats is the starting point for considering the investment in data mesh. Sufficient time and money for the journey and a culture that can embrace distributed decision-making are also key considerations for enduring success with data mesh.
If you determine that data mesh is a good fit for your organization, what’s next? To find out, look for my next blog, in which I’ll cover 10 data mesh best practices.
- The Four Pillars of Data Mesh
- Who is Data Mesh For?
- Conclusion