Why Cloud Data Governance is Critical: 9 Key Principles
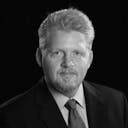
By John Wills
Published on November 11, 2021
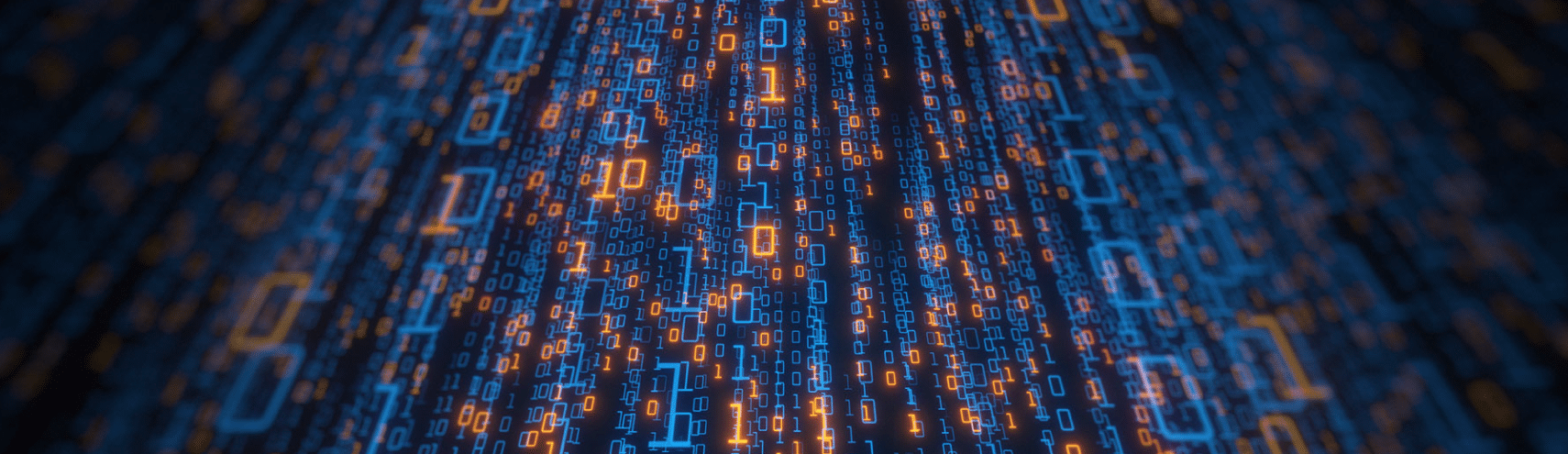
Today’s organizations are rapidly embracing the cloud. This is mostly due to cost-saving and data sharing benefits. As IT leaders oversee migration, it’s critical they do not overlook data governance. Data governance is essential because it ensures people can access useful, high-quality data. Therefore, the question is not if a business should implement cloud data management and governance, but which framework is best for them.
Whether you’re using a platform like AWS, Google Cloud, or Microsoft Azure, data governance is just as essential as it is for on-premises data. Let’s take a look at some of the key principles for governing your data in the cloud:
What is Cloud Data Governance?
Cloud data governance is a set of policies, rules, and processes that streamline data collection, storage, and use within the cloud. This framework maintains compliance and democratizes data. It enables collaboration, even as your data landscape grows larger and more complex.
Active data governance improves efficiency, minimizes security risks, and improves the quality and usability of data. The cloud environment adds unique layers of complexity around cybersecurity and access. For instance, the cloud needs security controls that address encryption, access controls, security groups, audit trails, and application access rules. These ensure data is protected and secure when traveling to and from the cloud. The good news is that data governance is evolving, and can be deployed to hybrid and multi-cloud environments.
9 Key Principles of Cloud Data Governance
Every business needs high-quality data. This requires a disciplined process that uses policies and standards. Ideally, that process is managed by a team of experts. Collectively that is a data governance program. Data governance is not new, but the rapid growth of the cloud has exposed older processes and demands new innovations. These 9 key functions are the most important:
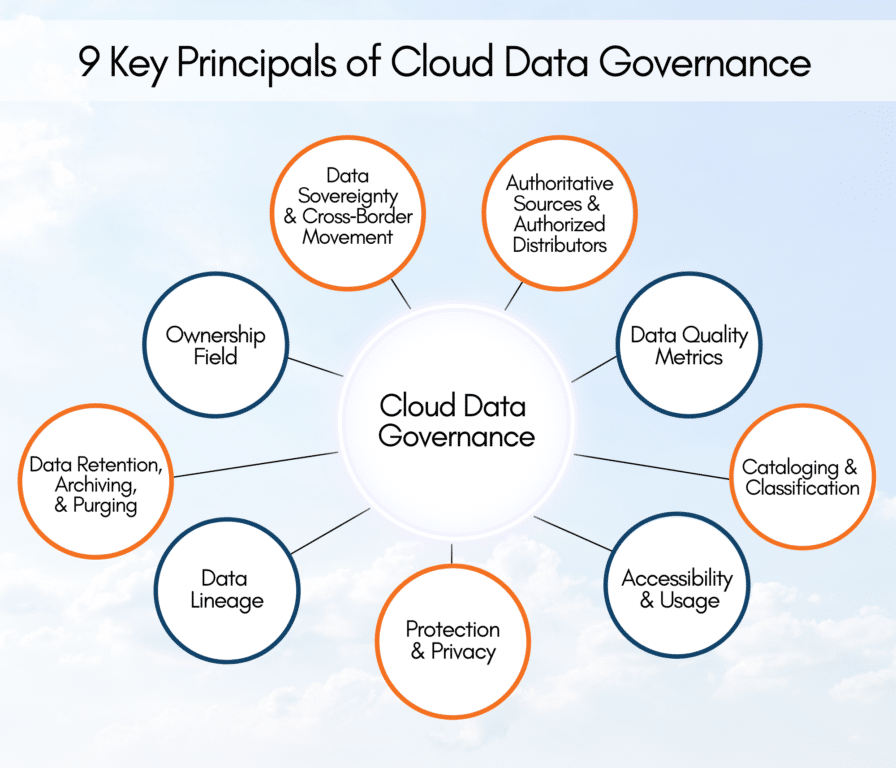
1. Authoritative Sources and Authorized Distributors
With the rise of the cloud the cost of storage is historically low and the number of people with skills needed to copy, transform, and move it is historically high. The result is an explosion of derivative data and very few people who understand its original origin. This can create a crisis of confidence and/or serious mistakes when incorrect data is used to draw conclusions and make business decisions.
What’s needed is the enforcement of authority. What data is the most trustworthy, and who has the authority to lay that claim? It’s not good enough to only declare the data as authoritative. The distributor of the data must also be authorized as a known, reliable source who is held to standards. This is especially important as it relates to the use of sensitive data and must apply to both the physical transfer of data as well as the inclusion of data in an algorithm, query, or aggregate result.
Achieving this is not easy. It requires assigning responsibility to individuals. They must maintain the accuracy of the authoritative designation and associated standards. Ultimately, this creates a framework of accountability. It’s the one thing that can save data teams from the risk of processing data from their own circular references, as this framework is a credible check-and-balance.
2. Data Sovereignty and Cross‐Border Movement
International data sharing is essential for many businesses. The free movement of data enables them to utilize common infrastructure to serve multiple markets, meaning that digital goods and services are spread to customers quickly and efficiently.
However, privacy regulations have emerged in the last few years — GDPR , CCPA in California, etc. — and simply sharing data across borders is not permitted. Any cross-border movement must adhere to different restrictions and compliance mandates with authorized supervision and approval. A Multi-Cloud Data Sharing Agreement, in which both the provider and consumer agree to the compliance mandates for their respective locations, solves this issue.
For instance, on modern data cloud platforms, like Snowflake, users can easily work with data while another cloud queries the data. This open access removes the need to share or send files. While Snowflake streamlines the cloud-data-sharing process, Alation secures cross-border movement and maintains compliance with a Multi-Cloud Data Sharing contract.
3. Cataloging & Classification
Modern data catalogs are far more than a metadata repository or your grandfather’s data dictionary. They continually analyze data and metadata to provide insight that enables data governance at scale. For example, a data catalog will identify metadata and structural changes in a data source and then automatically perform discovery, profiling, and classification tagging. It may also trigger notifications, workflows, and other actions based on the tagging.
In addition, a data catalog provides deep and rich attributes, such as business, technical and compliance classifiers, association to governance policies, as well as data source-specific access and security policies.
The modern data catalog is the foundation upon which a governance framework is implemented. Today, with the proliferation of multi-cloud, hybrid, and on-premise architectures, a data catalog is more important than ever. As data volume and complexity grows, a data catalog serves as the one place where all data may be classified — and governed accordingly.
4. Accessibility & Usage
Not just anyone in your organization should have access to sensitive data. If access to data is not sufficiently controlled, your business could experience data leakage, corruption of data, reputational damage, or criminal manipulation of business processes. Consequently, this data is then at risk of not being fit for purpose.
Access and usage of sensitive data begins with policies. High-level policies communicate requirements; these must be expanded on to include standards specific to cloud data sources. These are essentially the rules/conditions that will be the responsibility of key people (stewards, security experts, auditors) to implement (using both manual and automated processes). Some of these standards will be implemented in specific technologies as entitlements, masking, encryption, profiling, etc. The relationship between these lower level technical implementations and the high-level policies provides a cross-enterprise view of how governance is actually being applied and enforced.
5. Protection & Privacy
Data loss or breaches of sensitive data will cost a business in regulatory fines, reputational damage, and legal action. To properly protect data, appropriate security controls must be in place to encrypt sensitive data. Security controls must be recorded within the data catalog for any and all sensitive data. This ensures security and compliance across all data in the cloud. It also reduces time and money spent on traditional manual processes.
Data Privacy Impact Assessments (PIA) are another way to maintain protection and privacy. PIA’s identify assets where you need deeper analysis, mainly for risk and liability reasons where an audit trail is necessary. PIA’s should be used as a proactive mechanism used to minimize risk exposure.
Policies and standards must be defined and used to proactively trigger the need for a PIA. These are based on conditions such as the security classification, geographic location data storage, lifecycle stage (sandbox, production). The standards must also define the expiration for PIA so a reevaluation is triggered.
6. Owners & Stewardship
Sensitive data should never be left unmanaged. If you’re managing data in the cloud and a piece of data has been marked sensitive, it must have an owner. An ownership field capability ensures that all sensitive data has an owner, and if it doesn’t, someone is alerted to remediate it. Solutions that provide this feature ensure that sensitive data always has an owner and compliance is maintained.
Ownership and stewardship are often confused. Stewards can be delegated the responsibility to govern data but they are not the owner and certain situations may call for them to escalate decisions. For instance, establishing a basic data-sharing agreement with a consuming party could be done by a steward, but a request for more expansive or frequent access to a data source may have to be negotiated and agreed on by the data owner.
7. Data Quality Metrics
Measurements of data quality are often the most used data governance metric. Data quality metrics enable data stewards to determine if data is fit for purpose. This valuable information should be visible to both data owners and consumers.
Data quality metrics allow users to see how many times a data set has been entered and how many data values are similar across systems. They also measure data accuracy, consistency, completeness, and integrity. Businesses quantify their data quality by identifying the number of data issues found and the number of dividends they gain by fixing those issues.
In short, a framework that focuses on data quality metrics will help to show the value of cloud data governance to senior management; thus improving overall workflow performance and transparency.
8. Data Retention, Archiving, and Purging
The data lifecycle must be planned and managed. This means that organizations should know where sensitive data exists, how long it should be kept, and its requirements. This is especially important for future audits. Failure to follow data-specific jurisdiction could result in legal action for your organization.
But complying with these requirements is hard, and even more so when the process is manual. Using automation to retain, archive, and purge data will significantly improve operational efficiency. It also ensures that data is in line with the legislative, regulatory, and policy requirements.
9. Data Lineage
Businesses must be able to trust the data that they use. Not only because data accuracy is important, but because the cost of producing incorrect data is significant.
For this reason, data lineage is a powerful tool for understanding data. Data lineage helps you determine data’s suitability for usage. It does this by sharing key details, like how it’s changed over time (if it’s been optimized or improved), and who was involved in the processing. With these clues, users can discover the source of errors.
Transparent lineage also ensures that information is available for all sensitive data. This starts by confirming that data has been sourced in a controlled manner and by generating a data lineage report for any specific data asset — which can all be done through automation. Using automation to track the lineage of a given asset streamlines data for reports and reduces costs of manual labor.
Drive Data Governance in the Cloud with Alation
Successful cloud data migration and management all stem from strong data governance. Data governance is essential for any organization in the cloud. And while there are many cloud data governance platforms out there, it’s important to focus on key attributes that allow you to find trusted data, meet governance requirements, and operationalize governance at scale.
Alation’s Active Data Governance allows you to do all of this and more. Alation’s robust cloud data governance capabilities encompass all of the key attributes and policies we just identified, as well as deep impact analysis, and data strategy that drives cloud strategy.
- What is Cloud Data Governance?
- 9 Key Principles of Cloud Data Governance
- 1. Authoritative Sources and Authorized Distributors
- 2. Data Sovereignty and Cross‐Border Movement
- 3. Cataloging & Classification
- 4. Accessibility & Usage
- 5. Protection & Privacy
- 6. Owners & Stewardship
- 7. Data Quality Metrics
- 8. Data Retention, Archiving, and Purging
- 9. Data Lineage
- Drive Data Governance in the Cloud with Alation
Contents
FAQs
Cloud data governance is a set of policies, rules, and processes that streamline data collection, storage, and use within the cloud. This framework maintains compliance and democratizes data. It enables collaboration, even as your data landscape grows larger and more complex.