The Value of Catalog-Led Data Governance
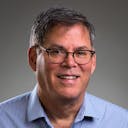
By Myles Suer
Published on November 4, 2021
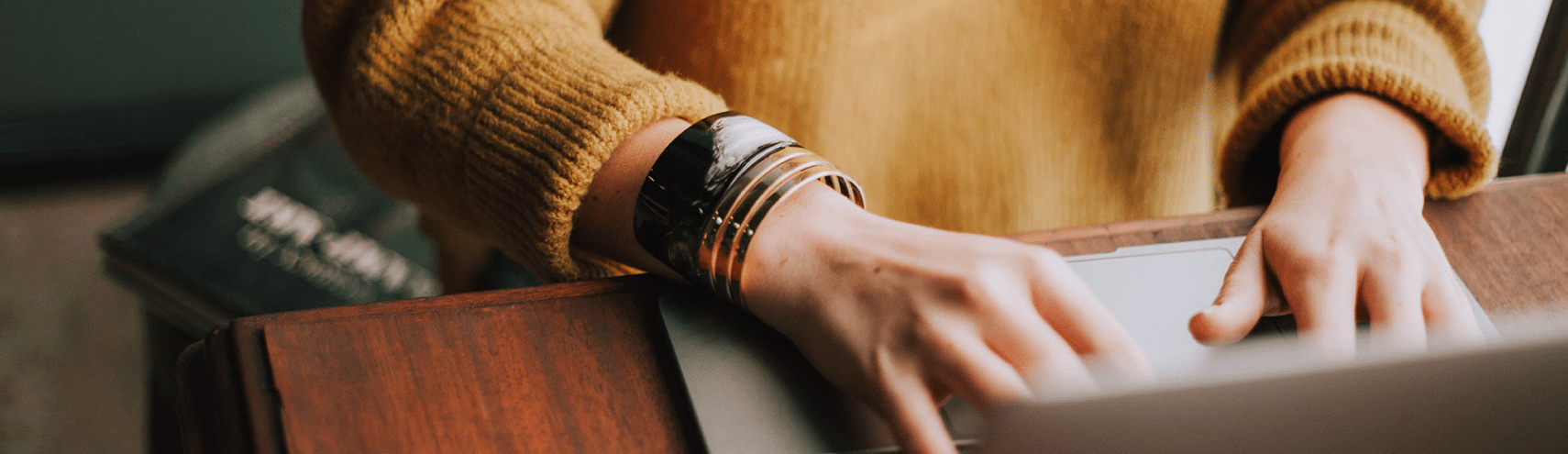
I don’t know about you, but every once in a while someone asks me a question and the answer completely changes my perspective on a topic. This happened for me a lot during my four startups. I liken it to walking into a room anew. I might know the room but everything in the room has changed with the new perspective.
This week I was talking to a data practitioner at a global systems integrator. The practitioner asked me to add something to a presentation for his organization: the value of data governance for things other than data compliance and data security. Now to be honest, I immediately jumped onto data quality. Data quality is a very typical use case for data governance.
However, I paused and asked myself: What is the value that customers of Alation actually got for non-compliance or data security use cases? To my surprise, the customer case studies largely centered around the value provided from self-service business intelligence.
The value of the data catalog depends on the audience. For data modelers, value arose from spending less time finding data and more time modeling data. For analysts, the catalog relieved them of the growing backlog of requests from end users for data. Analysts also saw an unquantified impact regarding the time to make a business decision. In fact, Alation announced an acquisition that will accelerate the availability of data to end users without programming skills.
At this point, I shared my analysis with a colleague, John Wills. John said that this makes the case for what should be called “catalog led data governance”. In other words, for data leaders, installing a data catalog first can establish the business value of data governance. They can then incrementally add data governance to the mix in order to add additional business value over time.
Why Catalog-Led Data Governance?
So let’s dig a bit deeper into this concept and why it makes so much business sense.
Historically, data practitioners have tried to mature their data processes in reverse order. Data governance focused on compliance and locking down data; only after this was accomplished did leaders focus upon “data monetization”.
Data monetization included activities such as enterprise alignment on common business terms, defining domains and taxonomies, conceptual/canonical enterprise data models with cross-walks to reference data, defining escalation paths for metadata changes, etc.
The problem is that these things provide little to no immediate value to the largest population of data consumers, represented by business analysts, managers, and line employees who are simply struggling with trying to find the right data to use and understand if they can trust it.
What this population needs is quite simple. They need to understand that there is a single repository (data catalog) that reflects the as-is state of the business in the form of available data, reports, and the identification of experts who can help them with both. Anyone who can consolidate this and allow them to see it in one place is going to be their hero.
Starting with this flips the equation and makes the governance team the provider of immediate value that everyone understands and can take advantage of. And once value is being delivered and adoption is moving rapidly, everyone becomes anxious for it to continually improve, which is where adding incremental governance capabilities comes in.
And as teams introduce additional metadata, such as classifications, expirations and quality scores, trust in the data grows. This doesn’t feel like a policing operation, but is correctly perceived as improving the ability for data consumers to be more effective with the use of data assets and reports. This continues as the governance team layers in business, technical, and compliance related metadata that enriches users’ understanding of the data and speed at which they can work.
This process builds a thriving community who is deriving what I call ‘core’ business value from a governance data catalog. Once this community has started to grow, it’s time for the enterprise architects to enter the equation to start working on cross-functional alignment. This includes the aforementioned alignment of business terms, cross-functional domains, master data definitions, reference data mapping, and much more. These are not easy, but the governance and data management teams have the benefits of a successful, on-going program that is providing ‘air cover’ and funding for the work.
Adding Data Governance When a Data Catalog Exists
Data governance should be based on 4 principles:
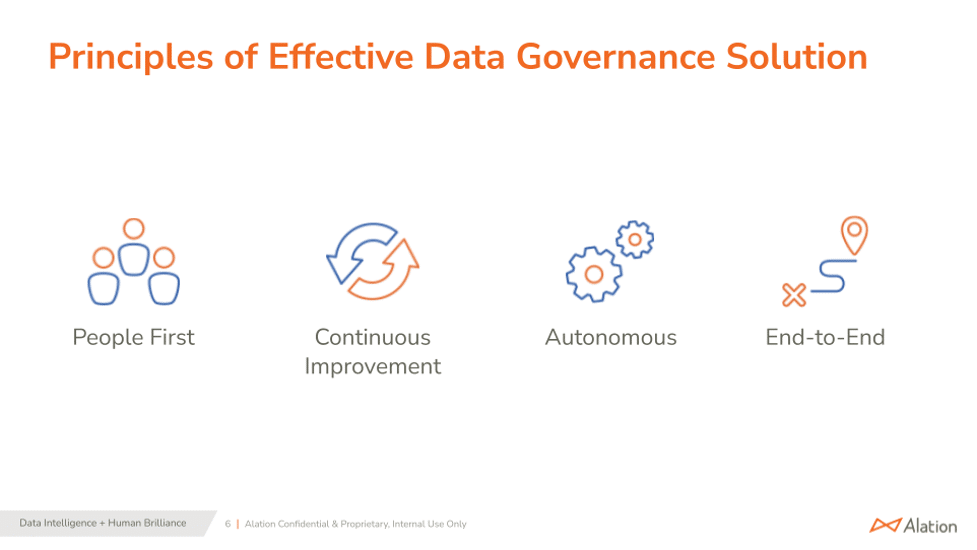
These principals work together. Implementing the catalog first is an important step in being people first. This is because the right data catalog can empower data democracy and the ability for people to use data to transform their jobs. You are assembling a cadre of data experts with the catalog. For this to work, you want a catalog that works largely autonomously and continuously learns via AI and machine learning (ML). With this, analytics can determine how well you are doing and where there are holes in your data.
So if you have an intelligent data catalog, what is left to implement to do the full monty of data governance?
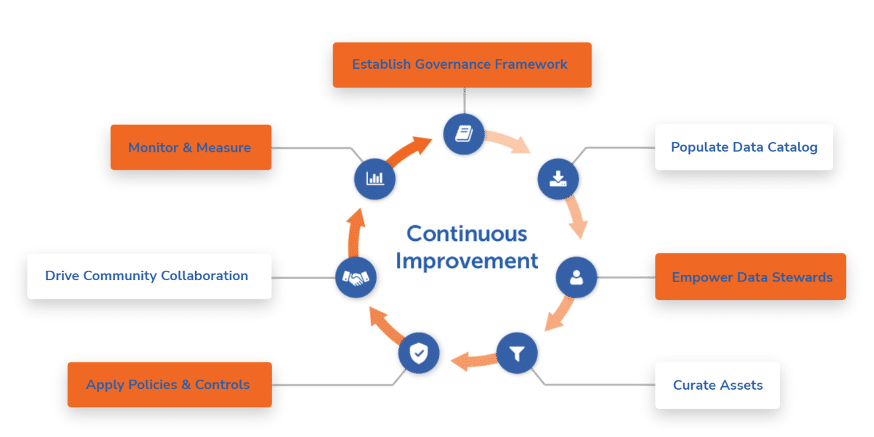
As you can see in the above data governance wheel, a lot of the heavy lifting is already done once you have implemented the data catalog. You only need to capture the data governance mission and vision and create overarching policies, standards, and glossaries. Next, you need to turn data experts into data stewards and automate a lot of the dog work via stewardship processes with the Stewardship Workbench, and the setting up of policies workflows.
With this, you can establish controls for the sensitive data you discover and monitor and measure how you are doing. Adding these four steps allows you to better control data quality, govern data access, and commit to data privacy. For those that are already Alation customers this is an easy move.
Parting Words
Obviously, there are organizations that will need to start the opposite way around. For them a data catalog built on AI/ML is a huge accelerant to launching an effective data governance program, as AI/ML can offload much of the manual burden of managing data access controls and privacy. Hopefully, catalog led data governance is a new way to think of your data processes.
- Why Catalog-Led Data Governance?
- Adding Data Governance When a Data Catalog Exists
- Parting Words